the Creative Commons Attribution 4.0 License.
the Creative Commons Attribution 4.0 License.
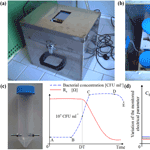
A portable sensor system for bacterial concentration monitoring in metalworking fluids
Carola Parolin
Beatrice Vitali
Bruno Riccò
The detection of bacterial concentrations in metalworking fluids (MWFs), oil-in-water emulsions used in the cutting industries for cooling and lubrication, is important in order to extend the product life-cycle and plan its disposal according to regulations and legislations. The standard method of measuring culturable bacterial concentration is the plate count technique (PCT) that, however, has long response times and is not suitable for automatic implementation outside a laboratory. In this paper a portable sensor system that measures the bacterial concentration in liquid and semi-liquid media exploiting impedance microbiology is presented and tested for the application of MWF microbial monitoring. A set of MWF samples, taken from metalworking plants, have been tested and good agreement has been found between the system response and that of the PCT. The proposed system allows automated bacterial concentration measurements with shorter response times than the PCT (4 to 24 h vs. 24 to 72 h) and is suitable for in-the-field MWF monitoring.
- Article
(1106 KB) - Full-text XML
- BibTeX
- EndNote
Bacterial concentration detection and monitoring are important in different fields (Bahadir and Sezginturk, 2015), such as food quality assurance (Fakruddin et al., 2013; Calix-Lara et al., 2014), clinical analysis (Singh et al., 2014) and environmental monitoring (Rizzo et al., 2013), in order to (a) ensure that the total bacterial concentration does not exceed thresholds set by national and international regulations, and (b) guarantee the absence of particular pathogens, such as Salmonella typhimurium and Escerichia coli O157:H7, representing a threat to human health even at very low concentrations.
Another interesting application is the contamination monitoring of metalworking fluids (MWFs) (Bakalova et al., 2007), namely oil-in-water emulsions used for cooling and lubrication in metalworking plants (Stephenson and Agapiou, 2005). Due to the presence of compounds such as glycols, fatty acid soaps and amines, bacteria can easily proliferate in MWFs, thus leading to product degradation (hence, loss of performance), as well as to potential threats to the health of exposed workers, since MWFs are dispersed in the air as aerosols during operation (Kriebel et al., 1997; Zacharisen et al., 1998). Once worn out, MWFs become a waste and must be disposed of according to regulations, using techniques such as membrane filtration (Cheryan and Rajagopalan, 1998), electrocoagulation (Kobya et al., 2008) and biological treatment (Van Der Gast et al., 2004). MWF disposal, then, represents a significant cost and has an impact on the environment. In order to extend the life of MWFs as much as possible, bacterial concentration must be regularly measured and counteractions (i.e. addition of a biocide to the product) must be taken when needed.
The standard method to measure culturable bacterial concentration is the plate count technique (PCT) (Grigorova and Norris, 1990) that is accurate and reliable but has long response times (in the range 24 to 72 h) and requires manual operations by trained personnel in a laboratory. Thus, it is not suitable for in situ measurements, nor as the base for automatic instruments.
Many alternatives to the PCT have been proposed in the literature, based on different transduction principles, such as turbidimetric (Koch, 1970), where the optical absorbance at a particular wavelength (usually 600 nm) is used to estimate bacterial concentration; electrochemical biosensing (Radhakrishnan et al., 2014), where a particular bio-receptor is immobilized on the sensing electrodes and the binding of the target bacterial strain produces changes in some electrical parameters; or flow cytometry (van Nevel et al., 2013), used in commercial instruments such as Bactoscan by Foss Electric.
Other culture-independent techniques have been implemented for bacterial concentration measurements and applied to MWFs. Such techniques include quantitative polymerase chain reaction (qPCR) (Saha and Donofrio, 2012), adenosine triphosphate (ATP) bioluminescence measurements (Webster et al., 2005) and matrix-assisted laser desorption ionization time of flight (MALDI-TOF) mass spectrometry measurements (Koch et al., 2015). All these are characterized by near-real-time measurements and can also detect non-culturable bacterial strains. However, qPCR and MALDI-TOF require expensive machineries and equipments, as well as highly trained personnel. ATP measurements have been characterized by low accuracy for a long time due to chemical interferences with the ingredients of MWFs. Recent advances indicate the possibility of improving the measurement accuracy by adding a filtration step (Canter, 2009) that, however, makes the procedure significantly more complex.
The industrial standard for in-the-field MWF bacterial monitoring is based on dip-slide test kits that are time-consuming (36 to 48 h for most bacterial species) and lack the accuracy of the PCT.
Another technique used to measure bacterial concentration is impedance microbiology (IM) (Firstenberg-Eden and Eden, 1984), exploiting the fact that bacterial metabolism transforms uncharged or weakly charged compounds into highly charged ones, thus producing a measurable change in the sample electrical parameters, allowing us to estimate the unknown bacterial concentration. IM can be easily implemented in automatic form and provides results in a shorter time compared to the PCT. IM has been successfully tested for bacterial concentration measurements in various types of samples, such as ice cream (Grossi et al., 2008, 2010), meat (Firstenberg-Eden, 1983), vegetables (Hardy et al., 1977), raw milk (Grossi et al., 2011a), fresh water (Grossi et al., 2013), beer (Pompei et al., 2012), and human urine samples (Settu et al., 2015), and to test the efficiency of various antibiotics and chemical preservatives (Zhou and King, 1995).
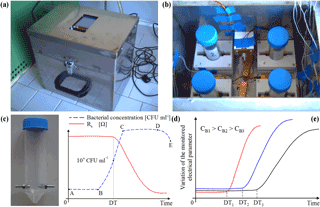
Figure 1Photographs of the outside (a) and inside (b) of the portable sensor system for bacterial concentration measurement. Modified vial used to host the sample (c). Measured sample resistance and bacterial concentration vs. time (d). Variation of the measured electrical parameter vs. time for samples featuring different bacterial concentrations (e).
In this work we present results obtained by testing a set of MWF samples using a new portable sensor system, based on IM. The investigation aims at determining the total bacterial concentration (hereafter simply called “bacterial concentration”) of all bacterial species present in the sample. The sensor could also be employed for the measurements of the concentration of a particular microbial species, by diluting the sample in a selective enriching medium, such as MacConkey Broth for coliforms or Mannitol Salt Broth for staphylococci. The proposed instrument, exploiting information and communication technology (ICT) solutions to measure, process and transmit data, can be used, by anybody, for in situ microbial screening inside metalworking plants.
The portable system, shown in Fig. 1a and b, is composed of an aluminium box (26 × 18 × 25 cm) that can host up to four different samples and two ad hoc designed electronic boards (based on STM32 microcontrollers) devoted to electrical measurement and thermoregulation, respectively (Grossi et al., 2017). Within the chamber, heating is realized by means of four power resistors (50 W, 1.2 Ω) connected in series. A NTC temperature sensor (B57045K produced by TDK) is placed inside the chamber to provide a feedback to the thermoregulation system. The samples under test (SUT) are contained in 50 mL polypropylene vials featuring two stainless steel electrodes (diameter 5 mm, spaced 12 mm) for electrical characterization (Fig. 1c). On the top cover of the box, a 320 × 240 LCD display and four buttons are used as a user interface. A USB port allows the measured data to be transferred to a PC for filing and further processing.
2.1 Working principle
Each SUT, possibly diluted in an enriching medium, is hosted in one of the vials placed inside the system chamber, heated to a chosen temperature (T∗), in our case T∗ = 37 ∘C to favour bacterial growth. After a time (τ = 60 min) needed for the temperature to stabilize, the SUT electrical parameters (i.e. resistive and capacitive components of the impedance) are measured at regular time intervals of 5 min. As shown in Fig. 1d, until the SUT bacterial concentration (CB) is lower than a critical threshold CTH, of the order of 107 colony forming unit (CFU) mL−1, the electrical parameters are essentially constant, while they deviate from the baseline value when CB > CTH. The time at which the electrical parameters start to deviate from their baseline values, denoted the detection time (DT), is a linear function of the logarithm of the initial (unknown) CB that can thus be estimated from DT. The curves of the monitored electrical parameter vs. time are shown in Fig. 1e in the case of three samples featuring different contaminations (CB1, CB2 and CB3, respectively). Of course, lower values of CB lead to higher DT. Plotting the measured DTs vs. the logarithm of corresponding values of CB, a calibration line can be obtained that allows us to estimate CB from the measured DT.
The calibration line can also be used to estimate parameters describing the bacterial growth kinetics, such as the lag time TLAG (i.e. the time needed for the bacteria to adapt to the growth medium) and the mean generation time TGEN (i.e. the mean time between cell duplication). In particular, for this purpose the following equations can be used (Grossi et al., 2009):
where A and B are the slope and offset of the calibration line, respectively, while τ is the delay needed for the thermal chamber to reach equilibrium.
If the frequency (f) of the applied test signal is lower than 1 MHz, the system composed of the SUT in direct contact with the electrodes can be modelled as the series of a resistance (Rs), accounting for the medium electrical conductivity, and a constant phase element (CPE), taking care of the capacitive electrode–electrolyte interface. Thus, the impedance Z can be modelled as
Q is the interface capacitance and α an empirical parameter accounting for the non-ideal behaviour of CPE (if α=1, CPE becomes an ideal capacitance).
2.2 Measurement of the electrical parameters
The SUT electrical parameters Rs and Q are measured by electrical impedance spectroscopy (EIS) (Grossi and Riccò, 2017a), a technique widely used also for human body analysis (Mialich et al., 2014; Khalil et al., 2014), food characterization (Harker and Maindonald, 1994; Grossi et al., 2011b, 2012a, 2014a, b), corrosion monitoring (Loveday et al., 2004; Bonora et al., 1995), battery management (Ran et al., 2010; Cuadras and Kanoun, 2009) and conductometric titrations (Grossi and Riccò, 2017b). A sine-wave voltage test signal Vin(t) is applied at the sensor electrodes,
and the current Iin(t) through the electrodes is measured by
where VM,in and IM,in are the voltage and current amplitudes, respectively, while φ is the phase difference between Vin(t) and Iin(t). The impedance Z is then calculated as
All electrical measurements are carried out by an ad hoc designed electronic board (based on the STM32L152RET6 microcontroller), and a simplified schematic of the measuring circuit is shown in Fig. 2. A sine-wave voltage signal VA(t) is generated using the 12-bit digital-to-analogue converter (DAC) of the microcontroller. The signal is then scaled with an inverting amplifier (ratio 1 : 10) to generate the VB(t) signal applied to the sensor electrodes. The current through the electrodes is fed to a current-to-voltage (I/V) converter that generates a voltage VC(t) proportional to the current. The I/V converter feedback resistance is a programmable digital potentiometer (MCP4131 127 steps 10 kΩ full range) to allow a wide range of impedances to be measured.
Two analogue multiplexers (ADG804YRMZ) allow up to four different SUTs to be tested in a single assay. The VA(t) and VC(t) signals are acquired by the 12-bit analogue-to-digital converter (ADC) of the microcontroller, the sine-wave parameters are calculated using the algorithm discussed in Grossi et al. (2012b) and the SUT impedance is calculated using the following equations:
where VM,A and VM,C are the amplitudes of VA(t) and VC(t), respectively; φ is the phase difference between VC(t) and VA(t); RF is the resistance of the digital potentiometer (controlled by the microcontroller via the SPI interface).
The impedance Z is measured for f = 100, 500 and 1000 Hz, while Rs and Q are calculated by fitting the measured data with the model of Eq. (3).
2.3 Chamber temperature control
The chamber thermoregulation board is based on the STM32F103 microcontroller that drives the power resistances using a PWM signal and a FODM1008R2 octocoupler (Fairchild) to isolate the board low-power and high-power sections from one another. The target temperature (T∗) inside the chamber is regulated using a PID algorithm (updated every 1 s) that modulates the duty cycle of the PWM signal (PWMDC) according to the formula
where Pk, Ik, and Dk are the proportional, integral, and derivative components of the PWM signal duty cycle at time k defined as
The temperature at time k (Tk) is measured with a voltage divider composed of a NTC temperature sensor and a 10 kΩ resistance. The output voltage is acquired by the microcontroller ADC and converted to the temperature value.
The PID parameters KP, KI and KD are chosen to quickly reach the steady state T∗ without excessive oscillations. In Fig. 3a the chamber temperature is plotted vs. time for three different sets of the PID parameters. The set KP=2, KI=0.003, KD=0 is found to provide the best performance, namely 30 min to reach the steady state and further oscillations smaller than 0.2 ∘C.
To investigate the temperature dynamics of the SUT, a test has been carried out where an additional temperature sensor has been placed inside the sensor vial in direct contact with the SUT. The results are shown in Fig. 3b, where the temperature in the chamber (external sensor) and that of the SUT (internal sensor) are plotted vs. time. As can be seen the SUT temperature is characterized by a much slower time response, reaching 74 and 89 % of the full variation after 60 and 120 min, respectively.
2.4 Microbiological analysis
Escherichia coli ATCC 11105 was cultured in Luria Bertani (LB) broth (Tryptone 10 g, yeast extract 5 g, NaCl 10 g in 1 L of distilled water) for 18 h, at 37 ∘C with vigorous agitation, to obtain an approx. 109 CFU mL−1 stock suspension. Decimal dilutions of the stock suspension were prepared in an enriching medium in the range 102–108 CFU mL−1, and tested in the sensor system (20 mL final volume). Three different media were employed: LB broth, modified Luria Bertani (MLB) broth (Tryptone 10 g, yeast extract 5 g in 1 L of distilled water), and nutrient broth (Difco nutrient broth 8 g in 1 L of distilled water). MWF samples were obtained from different metalworking plants in Bologna (Italy) and diluted in the enriching media in the ratio 1 : 10. MWF samples feature an oil concentration between 1 and 10 %, with a microbial population composed of different species and strains. These samples have been used in metalworking plants for different times and feature different levels of contamination and usage. Once obtained from metalworking plants, each sample was stored at environmental temperature before testing. The bacterial concentration of culturable cells (hereafter simply bacterial concentration) was determined by the PCT immediately before the start of each assay.
The PCT was performed by plating decimal dilution in physiological solution (NaCl 9 g in 1 L of distilled water) of each sample onto LB or nutrient agar plates (LB or nutrient broth added with 1.5 % agar). Plates were incubated for 24/48 h at 37 ∘C. Plate count tests were performed in duplicate and the results were reported as CFU mL−1.
The system presented in this work has been tested with both control samples and real MWF samples obtained from metalworking plants in the industrial area near Bologna (Italy). In both cases, results from the sensor system, i.e. measured DTs for two electrical parameters (Rs and Q) and three different enriching media, have been compared with the bacterial concentration obtained with the PCT. For each medium, SUTs featuring different bacterial concentrations have been tested and the calibration line, TGEN and TLAG have been calculated.
Table 2Electrical parameter baseline values and variations induced by bacterial metabolism for laboratory prepared control samples in three different enriching media.

First, control samples obtained by inoculating different concentrations of a laboratory cultured strain of Escherichia coli in sterile enriching media have been tested, in order to check the system functionality. DT values have been registered for any experimental condition (dilution/medium) and compared to bacterial concentration measured by the PCT. Table 1 shows the calculated TGEN, TLAG and coefficient of determination R2 (qualifying the fit between measured data and calibration line) for the three tested media and for the Rs and Q electrical parameters; Table 2 presents the baseline values (Rs,baseline and Qbaseline) and the variations induced by bacterial metabolism (ΔRs and ΔQ) for the three tested media. In the case of the LB medium, only Q has been considered since Rs provides no reliable results due to the medium high electrical conductivity hindering conductivity variations due to bacterial metabolism. The MLB medium features lower accuracy in the estimation of bacterial concentration (lower values of R2). The calculated value of TGEN is in the range 17 to 25 min for all tested media and monitored parameters (as expected from the literature), with LB giving the best results in terms of sensor response time (lowest value of TGEN). As shown in Table 2, bacterial metabolism induces much stronger variations on Q than on Rs.
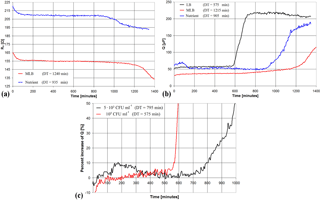
Figure 4Measured electrical parameters, Rs (a) and Q (b), plotted vs. time for a MWF sample featuring a contamination of 105 CFU mL−1. Percent increase in Q vs. time for two MWF samples featuring different bacterial concentrations in the LB medium (c).
Since measurements on the control samples have confirmed the functionality of the proposed system, hereafter all the investigations are carried out on real MWF samples. A set of 16 MWF samples, obtained from different metalworking plants, has been tested by diluting the sample in the enriching medium in the ratio 1 : 10. All measurements have been carried out in duplicate using the three enriching media mentioned earlier. In Fig. 4a and b the measured Rs and Q are plotted vs. time for a MWF sample featuring a bacterial concentration of 105 CFU mL−1, taken as an example. The Rs curve for LB is not shown since, due to the (too) high conductivity of the medium, no detectable variation is produced by bacterial metabolism. The results obtained with the MWF samples confirmed that LB is the enriching medium providing the fastest response and the best correlation with the PCT. Thus, in the following only results obtained with LB and parameter Q are shown.
Figure 4c shows the percent variation of Q vs. time for two different SUTs featuring different bacterial concentrations (5 × 103 and 105 CFU mL−1). As expected, the higher bacterial concentration leads to a lower value for DT (575 min) compared with the other case (795 min).
For each SUT the measured DT has been plotted vs. the bacterial concentration measured with the PCT. The results, shown in Fig. 5, have been analysed with the EXCEL XLSTAT add-on package software, and a linear regression (R2 = 0.9286) was found between DT and the logarithm of bacterial concentration, expressed as
where, assuming a confidence interval of 95 %, the slope of the calibration line is in the range −85.411 to −61.985, while the intercept falls in the interval 1351.716 to 1622.701.
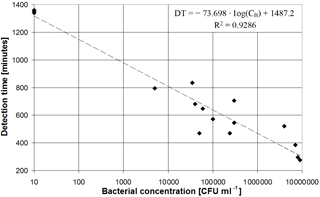
Figure 5Scatter plot of measured detection time vs. bacterial concentration measured by the PCT for all tested MWF samples.
Data variability has been investigated by analysing the measured DT for repeated assays carried out on the same sample.
For this purpose seven SUTs (characterized by DTs from 475 to 820 min) have been used, each tested twice. The average difference in these couples of measurements was 22.86 min, corresponding to a standard deviation of 14.39 min. According to Eq. (13), this results in an average difference for Log(CB) of only 0.31, clearly indicating that data variability is essentially due to the diversity of the bacterial microflora, while measurement repeatability plays only a minor role.
The kinetics of the population growth, calculated according to Eqs. (1) and (2), features TGEN in the range 42.96 to 59.2 min, and TLAG between 35.06 and 683.63 min.
As can be seen, these parameters have higher values and much higher dispersion than when a laboratory cultured single strain of Escherichia coli is used. This can be due to the large diversity of bacterial species present in the samples (higher dispersion) and the fact that autoctone bacterial cells need some time to adapt to the new growth medium (higher values of TGEN and TLAG).
The obtained calibration line has been used to estimate the bacterial concentration based on the measured DT. Figure 6 reports the scatter plot of the estimated bacterial concentration (CB) vs. the bacterial concentration measured by the PCT (CPCT), showing the linear regression line (Y=0.9999X) as well as the 95 % upper and lower bounds. The random variable Log10(CB∕CPCT), accounting for the difference between CB and CPCT, has been fitted with a Gaussian distribution using a Chi-square goodness of fit test with a significance level α=0.05. The computed p-value (0.237) is significantly higher than the significance level (0.05), thus confirming the hypothesis that Log10(CB∕CPCT) follows a Gaussian distribution. The random variable features an average value of −7.64 × 10−5 and a standard deviation (due to the diversity of bacterial species) of 0.563. According to the fitting distribution, in about 93.75 % of the cases bacterial concentration is estimated with an error lower than an order of magnitude (i.e. < 1); thus, the SUTs can be reliably clustered according to their level of contamination.
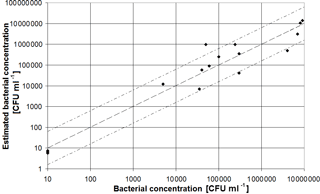
Figure 6Scatter plot of estimated bacterial concentration vs. bacterial concentration measured by the PCT for all tested MWF samples.
Overall, considering a critical threshold for bacterial concentration of 105 CFU mL−1 (a common value assumed in metalworking industries to discriminate low from high contaminations), the time response of the proposed system is less than 50 % of the time required by the PCT or dip-slide test kits. This allows us to carry out the measurements overnight and to obtain the response in the morning of the next day. By setting a DT threshold (DTTH) of 710 min, all samples featuring a bacterial concentration > 105 CFU mL−1 are correctly classified as contaminated (DT < DTTH), while those with bacterial concentration < 4 × 104 CFU mL−1 are classified as non-contaminated (DT > DTTH). The time response of the proposed system is longer than that of near-real-time assays, such as ATP bioluminescence. However, (a) sample preparation is much simpler (comparable to the dip-slide test kit); (b) it allows the detection of viable cells only; and (c) the correlation with the PCT is better (R2 = 0.9286 vs. R2 in the range 0.743 to 0.893 for ATP) (Webster et al., 2005).
This paper presents a portable sensor system, exploiting impedance microbiology, that can be used to measure bacterial concentrations in metalworking fluids (MWFs). The system, featuring a thermal chamber and two electronics boards, has been tested, and the results show that bacterial concentration can be estimated with good accuracy and that the samples can be clustered according to their level of contamination. Recently, many culture-independent techniques have been used to detect and quantify microbial species in MWFs. They have the advantage of real-time measurements and high performance but require expensive instruments and highly trained personnel. In addition, culture-independent methods do not discriminate live from dead microbial cells.
The system presented in this work can be used for quick (a few hours) and automated monitoring of MWF contamination, allowing reliable in situ detection, without the need for qualified personnel. The possibility of easy employment of the system can provide benefits to metalworking industries in terms of MWF extended lifetime, lower costs and reduced impact on the environment.
The underlying measurement data are not publicly available, but can be requested from the authors if required.
The authors declare that they have no conflict of
interest.
Edited by: Anita Lloyd Spetz
Reviewed by: two
anonymous referees
Bahadir, E. B. and Sezginturk, M. K.: Applications of commercial biosensors in clinical, food, environmental, and biothreat/biowarfare analyses, Anal. Biochem., 478, 107–120, 2015.
Bakalova, S., Doycheva, A., Ivanova, I., Groudeva, V., and Dimkov, R.: Bacterial microflora of contaminated metalworking fluids, Biotechnology and Biotechnological Equipment, 4, 437–441, 2007.
Bonora, P. L., Deflorian, F., and Fedrizzi, L.: Electrochemical impedance spectroscopy as a tool for investigating underpaint corrosion, Electrochim. Acta, 41, 1073–1082, 1995.
Calix-Lara, T. F., Rajendran, M., Talcott, S. T., Smith, S. B., Miller, R. K., Castillo, A., Sturino, J. M., and Taylor, T. M.: Inhibition of Escherichia coli O157:H7 and Salmonella enterica on spinach and identification of antimicrobial substances produced by a commercial Lactic Acid Bacteria food safety intervention, Food Microbiol., 38, 192–200, 2014.
Canter, N.: New test method for detecting microbes in MWFs, Tribol. Lubr. Technol., 65, 8–9, 2009.
Cheryan, M. and Rajagopalan, N.: Membrane processing of oily streams. Wastewater treatment and waste reduction, J. Membrane Sci., 151, 13–28, 1998.
Cuadras, A. and Kanoun, O.: SoC Li-ion battery monitoring with impedance spectroscopy, Proceedings of the 6th International Multi-Conference on Systems, Signals and Devices, 1–5, 2009.
Fakruddin, Md., Bin Mannan, K. S., and Andrews, S.: Viable but nonculturable bacteria: food safety and public health perspective, ISRN Microbiology, New York, USA, 2013.
Firstenberg-Eden, R.: Rapid estimation of the number of microorganisms in raw meat by impedance measurement, Food Technol., 37, 64–70, 1983.
Firstenberg-Eden, R. and Eden, G.: Impedance Microbiology, Wiley, New York, 1984.
Grigorova, R. and Norris, J. R.: Methods in micro-biology, Academic Press London, 22, 497–531, 1990.
Grossi, M. and Riccò, B.: Electrical impedance spectroscopy (EIS) for biological analysis and food characterization: a review, J. Sens. Sens. Syst., 6, 303–325, https://doi.org/10.5194/jsss-6-303-2017, 2017a.
Grossi, M. and Riccò, B.: An automatic titration system for oil concentration measurement in metalworking fluids, Measurement, 97, 8–14, 2017b.
Grossi, M., Lanzoni, M., Pompei, A., Lazzarini, R., Matteuzzi, D., and Riccò, B.: Detection of microbial concentration in ice-cream using the impedance technique, Biosensors and Bioelectronics, 23, 1616–1623, 2008.
Grossi, M., Pompei, A., Lanzoni, M., Lazzarini, R., Matteuzzi, D., and Riccò B.: Total bacterial count in soft-frozen dairy products by impedance biosensor system, IEEE Sens. J., 9, 1270–1276, 2009.
Grossi, M., Lanzoni, M., Pompei, A., Lazzarini, R., Matteuzzi, D., and Riccò, B.: An embedded portable biosensor system for bacterial concentration detection, Biosensors and Bioelectronics, 26, 983–990, 2010.
Grossi, M., Lanzoni, M., Pompei, A., Lazzarini, R., Matteuzzi, D., and Riccò, B.: A portable biosensor system for bacterial concentration measurements in cow's raw milk, Proceedings of the 4th IEEE International Workshop on Advances in Sensors and Interfaces, 132–137, 2011a.
Grossi, M., Lazzarini, R., Lanzoni, M., and Riccò, B.: A novel technique to control ice cream freezing by electrical characteristics analysis, J. Food Eng., 106, 347–354, 2011b.
Grossi, M., Lanzoni, M., Lazzarini, R., and Riccò, B.: Automatic ice-cream characterization by impedance measurements for optimal machine setting, Measurement, 45, 1747–1754, 2012a.
Grossi, M., Lanzoni, M., Lazzarini, R., and Riccò, B.: Linear non iterative sinusoidal fitting algorithm for microbial impedance biosensor, Sensors & Transducers Journal, 137, 235–244, 2012b.
Grossi, M., Lazzarini, R., Lanzoni, M., Pompei, A., Matteuzzi, D., and Riccò, B.: A portable sensor with disposable electrodes for water bacterial quality assessment, IEEE Sens. J, 13, 1775–1782, 2013.
Grossi, M., Di Lecce, G., Gallina Toschi, T., and Riccò, B.: A novel electrochemical method for olive oil acidity determination, Microelectronics Journal, 45, 1701–1707, 2014a.
Grossi, M., Di Lecce, G., Gallina Toschi, T., and Riccò, B.: Fast and accurate determination of olive oil acidity by electrochemical impedance spectroscopy, IEEE Sens. J, 14, 2947–2954, 2014b.
Grossi, M., Parolin, C., Vitali, B., and Riccò, B.: Bacterial concentration detection using a portable embedded sensor system for environmental monitoring, Proceedings of the 7th IEEE International Workshop on Advances in Sensors and Interfaces, 246–251, 2017.
Hardy, D., Kraeger, S. J., Dufour, S. W., and Cady, P.: Rapid detection of microbial contamination in frozen vegetables by automated impedance measurements, Appl. Environ. Microbiol., 34, 14–17, 1977.
Harker, F. R. and Maindonald, J. H.: Ripening of nectarine fruit (changes in the cell wall, vacuole, and membranes detected using electrical impedance measurements), Plant Physiol., 106, 165–171, 1994.
Khalil, S. F., Mohktar, M. S., and Ibrahim, F.: The theory and fundamentals of bioimpedance analysis in clinical status monitoring and diagnosis of diseases, Sensors, 14, 10895–10928, 2014.
Kobya, M., Ciftci, C., Bayramoglu, M., and Sensoy, M. T.: Study on the treatment of waste metal cutting fluids using electrocoagulation, Sep. Purif. Technol., 60, 285–291, 2008.
Koch, A. L.: Turbidity measurements of bacterial cultures in some available commercial instruments, Anal. Biochem., 38, 252–259, 1970.
Koch, T., Passman, F., and Rabenstein, A.: Comparative study of microbiological monitoring of water-miscible metalworking fluids, Int. Biodeter. Biodegr., 98, 19–25, 2015.
Kriebel, D., Sama, S. R., Woskie, S., Christiani, D. C., Eisen, E. A., Hammond, S. K., Milton, D. K., Smith, M., and Virji, M. A.: A field investigation on the acute respiratory effects of metal working fluids. I. Effects of aerosol exposure, Am. J. Ind. Med., 31, 756–766, 1997.
Loveday, D., Peterson, P., and Rodgers, B.: Evaluation of organic coatings with electrochemical impedance spectroscopy – part 2: application of EIS to coatings, JCT Coatings Tech, 1, 88–93, 2004.
Mialich, M. S., Faccioli Sicchieri, J. M., and Alceu, A. J. J.: Analysis of body composition: a critical review of the use of bioelectrical impedance analysis, International Journal of Clinical Nutrition, Vol. 2, 1–10, 2014.
Pompei, A., Grossi, M., Lanzoni, M., Perretti, G., Lazzarini, R., Riccò, B., and Matteuzzi, D.: Feasibility of lactobacilli concentration detection in beer by automated impedance technique, MBAA Technical Quarterly, 49, 11–18, 2012.
Radhakrishnan, R., Suni, I. I., Bever, C. S., and Hammock, B. D.: Impedance biosensors: applications to sustainability and remaining technical challenges, ACS Sustai. Chem. Eng., 2, 1649–1655, 2014.
Ran, L., Junfeng, W., Haiying, W., and Gechen, L.: Prediction of state of charge of lithium-ion rechargeable battery with electrochemical impedance spectroscopy theory, Proceedings of the 5th IEEE Conference on Industrial Electronics and Applications, 684–688, 2010.
Rizzo, L., Manaia, C., Merlin, C., Schwartz, T., Dagot, C., Ploy, M. C., Michael, I., and Fatta-Kassinos, D.: Urban wastewater treatment plants as hotspots for antibiotic resistant bacteria and gene spread into the environment: A review, Sci. Total Environ., 447, 345–360, 2013.
Saha, R. and Donofrio, R. S.: The microbiology of metalworking fluids, Appl. Microbiol. Biot., 94, 1119–1130, 2012.
Settu, K., Chen, C. J., Liu, J. T., Chen, C. L., and Tsai, J. Z.: Impedimetric method for measuring ultra-low E. coli concentrations in human urine, Biosensors and Bioelectronics, 66, 244–250, 2015.
Singh, R., das Mukherjee, M., Sumana, G., Gupta, R. K., Sood, S., and Malhotra, B. D.: Biosensors for pathogen detection: A smart approach towards clinical diagnosis, Sensors Actuat. B, 197, 385–404, 2014.
Stephenson, D. A. and Agapiou, J. S.: Metal Cutting Theory and Practice, CRC Press, Florida, USA, 2005.
Van Der Gast, C. J., Whiteley, A. S., and Thompson, I. P.: Temporal dynamics and degradation activity of an bacterial inoculum for treating waste metal-working fluid, Environ. Microbiol., 6, 254–263, 2004.
van Nevel, S., Koetzsch, S., Weilenmann, H.-U., Boon, N., and Hammes, F.: “Routine bacterial analysis with automated flow citometry, J. Microbiol. Meth., 94, 73–76, 2013.
Webster, A. R., Lee, J., and Deininger, R. A.: Rapid assessment of microbial hazards in metalworking fluids, J. Occup. Environ. Hyg., 2, 213–218, 2005.
Zacharisen, M. C., Kadambi, A. R., Schlueter, D. P., Kurup, V. P., Shack, J. B., Fox, J. L., Anderson, H. A., and Fink, J. N.: The spectrum of respiratory disease associated with exposure to metal working fluids, J. Occup. Environ. Med., 40, 640–647, 1998.
Zhou, X. and King, V. M.: An impedimetric method for rapid screening of cosmetic preservatives, J. Ind. Microbiol. Biot., 15, 103–107, 1995.