the Creative Commons Attribution 4.0 License.
the Creative Commons Attribution 4.0 License.
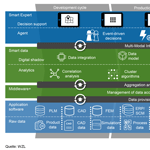
Sensor information as a service – component of networked production
Robert H. Schmitt
Christoph Voigtmann
Metrology has a key position in networked, adaptive production, with the task of a holistic and valid assessment of the state of various production scenarios. With the diminishing focus on a device-specific development towards an adaptive production network, which is less hierarchical in the sense of the “Internet of production”, and with the focus on the properties of cyber-physical systems (CPSs), new opportunities for the strengthening of metrology arise. Characteristic of these CPSs are sensors for multi-modal data acquisition, actuators for interaction with the environment, distributed computing power and the ability to spontaneously or permanently network itself. They form the basis for the creation of a “digital shadow” and thus are essential components of a model for process control. Current trends and challenges for metrology in networked production, such as multi-sensor systems, model-based measurements, virtual measurement processes or the integration into adaptable production systems, broaden the boundaries of future requirements of metrology, in particular with regard to its flexibility, speed and compatibility. A prerequisite is a scalable, specifiable information fusion. A solution to this is the service-based provision of sensor information, measurement data and decisions, which can be flexibly adapted to task-specific requirements. For this concept of “sensor information as a service”, development stages and prerequisites for its implementation as well as affected areas are discussed.
The core of the concept of Industry 4.0 is the connection of digitized products and production resources based on cyber-physical systems in real time (Bauernhansl et al., 2017). With the development of products and production resources towards intelligent systems and their connection to the “Internet of things” (IoT), services, methods and processes coming from the information technology sector are becoming increasingly important in the production environment (Bauernhansl et al., 2017; Brecher et al., 2017, 2014; Monostori, 2014). This trend goes hand in hand with the so-called “Internet of production” with its paradigm shift towards the focus on data-centric business models to secure competitive advantages (Brecher et al., 2017). This paradigm shift is based on several factors: on the one hand on cost-effective high-performance sensors and thus on a strongly increasing number of recorded production data. On the other hand, it is based on steadily decreasing costs for storage space and thus an increased number of available historical production data. In addition, the available computing power is exponentially increasing, enabling simple and fast data processing, data analysis and feedback of the results into production. The Internet of production focuses on real-time reliable and secure availability of information at any time and any place to enable systematic learning from the data collected for decision support. A schematic infrastructure of the Internet of production is shown in Fig. 1. The main driver of these three developments is the market of consumer electronics (Bauernhansl et al., 2017; World Economic Forum, 2017).
When comparing the digitization of different sectors, recurring trends and development stages can be identified, which can be represented, for example, by the so-called “digital maturity level” (O'Leary, 2009; Fenn and Linden, 2017; Schuh et al., 2017). This maturity assessment covers all areas of the company: from the degree of digitization of business processes to the management of IT processes, IT resources and IT technologies, to the assessment of a digital strategy and market development. A development that has just entered the field of production technology is “cloud manufacturing” (Wu et al., 2013; Xu, 2012; Soyata, 2015). Wu et al. (2013) define the concept of cloud manufacturing as a customer-centric production model that uses demand-based access to a shared network of different and distributed production tools to create temporary and reconfigurable production lines. Part of this development, which results from the low cost of data transfer, is the provision of customized services managed in the cloud and sold as a service. It is called “everything as a service” (XaaS) (Yang and Tate, 2012; Duan, 2012; Duan et al., 2015). A typical example is the provision of software without the user having to own or install the software, but only needing to access the software via the Internet (“software as a service”, SaaS). In the case of cloud-based services, this development is continuing, with the result that not only the software is offered as a service, but also, for example, the required infrastructure, hardware or entire platforms (“infrastructure as a service”, “platform as a service”). An overview of the current manifestations of the concept of everything as a service can be found in Duan et al. (2015).
The central questions of this article therefore are as follows. To what extent can the concept of everything as a service be transferred to metrology in a production environment? Which potentials result from this, and what could a possible design look like?
To answer these questions, it is worthwhile looking at current challenges and trends in metrology in networked production and related developments (Sect. 2). It is then explained what is meant by the term “sensor information as a service” and what these services can look like (Sect. 3). Based on this, necessary and expected developments are discussed, which are to be pursued for the implementation of the service concepts in metrology (Sect. 4).
The shift towards a digitized production influences the role of metrology within production. Current trends in production technology demand the further development of metrology, a process that can be described quite well with the terms “measuring in the model”, “model-based measurement processes”, “model-based process control” and “cyber-physical production systems” (CPPSs). The introduction of the “service” concept to metrology can be an answer to these challenges and can be seen as a complement to the presented trends.
An overview of the challenges and trends in metrology has already been provided by Imkamp et al. (2016, 2012), as well as by Schütze and Helwig (2017), and serves as a basis for the following overview. The key challenges for measurement technology can be described as faster, more accurate, more secure, more flexible and more holistic, faster meaning a requirement for higher process cycle times which can be achieved by increased integration, a reduction of measurement times or automated data processing. The demand for more accurate metrology results from the trend towards smaller tolerances and higher manufacturing precision in the production and is decisively reflected in the requirements for measurement deviations and measurement uncertainty, meaning the systematic and random deviations of measurement results as described in the Guide to the expression of uncertainty in measurement (JCGM, 2008). The demand for safety in metrology arises mainly from an increasing need to meet legal accountability obligations as well as an increasing need for validity of the acquired data. Validity in this context means that the data are available and correct and were acquired within required measurement uncertainty limits. The term flexible describes the demand for a cost-minimized adaptation of measurement technology to changing measurement tasks (different products, measures, accuracies, etc.). A field of application in which this requirement arises is the flexible use of measurement technology for micro and macro navigation, i.e., the generation of 3-D models in a production environment for the spatial positioning and orientation of production means (or their components) and workpieces under the restrictions of the uncertainty of the model and available calculation time. It is not evaluated in “classical” categories, but rather in a way that the “digital shadow”, meaning an application-specific image of the production state, can be used to control the production. For the planning of robot movements, for example, only rough planning and monitoring using metrology with relatively large permissible uncertainties is necessary in order to navigate within the target area. For the positioning at the target point and in order to exactly determine the deviation between the real and desired position, a fine-tuning and control of the movement by measurement systems with a lower uncertainty is necessary. This dynamic change in the requirement for measurement uncertainty during the process can, for example, be met by using additional, external measurement systems and including them into the metrological reference frame (Schmitt et al., 2014; Forbes et al., 2013; Wang et al., 2011; Ghidary et al., 1999; Li et al., 2013; Montavon et al., 2017). As a result, the role of production metrology changes away from the acquisition and connection of individual characteristics towards a holistic description of the products using additional data from the entire product life cycle.
2.1 Metrology architecture for cyber-physical production systems (CPPSs)
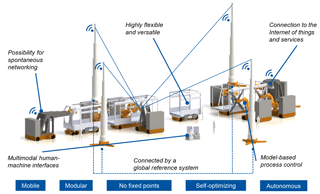
Figure 2Characteristics of a cyber-physical production system (CPPS) using the example of a free-chain assembly scenario. This figure is translated from Schmitt and Voigtmann (2017).
The use of cyber-physical systems (Monostori, 2014; Brecher et al., 2014; Rajkumar et al., 2010; Broy, 2010), their interconnection and the modularization of services as well as the encapsulation of subprocesses will make production even more flexible. The future of production as a CPPS is designed for individual production as well as a flexible adaptation of production to the requirements of the customer (Monostori, 2014; Monostori et al., 2016). The basic characteristics of a CPPS are given according to the working group Industry 4.0 (Brecher et al., 2017, 2014):
-
data acquisition via production-integrated sensors and measurement systems in real time,
-
storage and evaluation of data for the purpose of modeling,
-
interaction of the physical, human and digital world (multimodal human–machine interfaces) by actuators and
-
connection via digital communication devices among themselves as well as with the Internet of things and services.
This concept, which is based on multi-modal metrology, allows parts, hardware and sensors to be monitored and controlled within the entire production area; see Fig. 2. Production engineering concepts, which combine the indisputable advantages of a synchronous production flow with the free-networked island production (which itself focuses on volatility and small batch production) in a “Beyond Toyota” approach, are based on a mobile and modular design of all components. One example is the concept of a free float flawless assembly (Huettemann et al., 2016). The goal is to make products, production resources and technologies available as required in the entire workspace (Huettemann et al., 2016; Brecher et al., 2017). For example, in the case of highly precise production steps, a plurality of sensors can therefore be used together or additional sensors can be driven to the workplace in order to ensure more reliable monitoring and control of the production step (Brecher et al., 2017).
The goal is an automated and autonomous process control, which collects the required information as needed. As a result of the continuous monitoring of the products and production processes, an expandable digital image of the product (“digital twin”), which is designed in product development and various application scenarios, and an application-specific image of the production state (digital shadow) are created across all production steps (Bauernhansl et al., 2017; Brecher et al., 2014; Spencer et al., 2004). In the future, this will enable the inspection of the product using the digital representation of the product. As a long-term outlook, it is to be assumed that separate inspection processes and corresponding procedures for the final inspection will be drastically reduced.
As a result of this trend towards distributed and connected measurement and production systems, further challenges arise for metrology. In order to enable the connection and communication between measurement and production equipment, different scales have to be homogenized, various hardware (sensors, machines, IT infrastructures) have to be combined and manufacturer-specific controls and visualizations have to be standardized. This is the only way to ensure a uniform data exchange and uniform analysis and interpretation of the data. The central challenge here is to define these uniform data formats and communication interfaces independent of the manufacturer. In particular the provision of metadata is of greater importance in order to be able to describe the data as specifically as possible and to be able to use the data universally. On the one hand data formats and interfaces must therefore be standardized; on the other hand they should be able to record data and associated metadata from all kinds of different measurement and production processes.
There are some industry and application-specific approaches to solve these challenges, but no internationally established standards. Current developments are the STEP data format for product data within the ISO 10303 series (ISO, 2018), the RAMI model for the architecture description of Industry 4.0 components (VDI, 2017; DIN, 2016; Löwen et al., 2017, 2016) and OPC-UA as a communication protocol for machine data (Candido et al., 2010; Hannelius et al., 2008; Montavon et al., 2017). Further approaches can be found in context-based data formatting (Perera et al., 2014a), self-describing data models (NetCDF 2017), a universal description of metadata (Guenther and Radebaugh, 2004; Koza, 2003) and especially adapted descriptions for sensor networks in Li et al. (2013), Qingping et al. (2014), Huang and Javed (2008), Goos et al. (2003), Compton et al. (2009), Barnaghi et al. (2009) and Kim et al. (2008).
2.2 Model-based process control
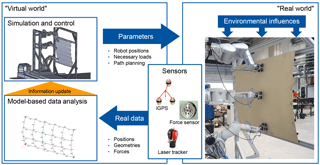
Figure 3Self-optimizing assembly of aircraft structures. This figure is translated from Schmitt and Voigtmann (2017).
If one follows this argument, it is to be expected that models for the feedback of the data with the aim of process control become more exact but at the same time more complex (Maropoulos et al., 2008, 2011; Pabst et al., 2010; Klocke et al., 2013; Schmitt et al., 2012; Möhring et al., 2010), since more and more process parameters and disturbance variables are considered in the process models for a more precise description and prognosis of the production processes. These parameters must be acquired and made available during operation. In addition, the quality of the data and their interpretation determines the quality and efficiency of the control. Hence, not only is it necessary to provide more data, but the data quality must also be ensured, meaning the completeness, consistency, timeliness and accuracy of the data which is required for its use. In combination with an increasing extent of monitoring and control of production processes, this leads to a high demand for valid, holistic process and status data. This requirement, however, can only be met by metrology. This is also known as information-rich metrology (Leach, 2016). Hence, in the future metrology will no longer be only necessary – in the metrological sense – for measurement and inspection tasks, but rather will play a dominant role in the production process with the provision of customer-tailored digital information. The current development of model-based process control will be illustrated by three examples. These examples have the fact that they use classical criteria of the inspection process capability as optimization parameters in a production-technological context in common.
An example of the model-based, metrology-assisted process control of production and assembly processes is the self-optimizing assembly of aircraft structures; see Fig. 3 (Permin et al., 2016; Schmitt et al., 2013a). The fulfillment of the measurement task is not the primary goal in this application, rather the fulfillment of the assembly task is. To optimize the assembly process, a model is created for the individual component-dependent geometric errors. The model allows compensation of geometrical errors during the assembly process. The compensation routine uses a large number of heterogeneous (sensor) data for this purpose. For this purpose, the pose of the robots and the part is recorded by means of iGPS and laser trackers, and the forces acting on the part, device and robots are recorded by means of strain gauges and load cells. The measurement technology serves as a link between the real and the virtual world. In addition, the CAD data and the robot control data are included. In this example, the challenge consists, on the one hand, of the modeling of the geometrical errors that has to be as realistic as possible, and, on the other hand, the homogenization of a wide range of sensor, plan and control data. A uniform definition of interfaces is essential for the modularization via services, in particular for the provision of the described sensor data as model input.
Another example of the use of complex process models in connection with metrology is the control of machine tools. By developing more comprehensive process models, the position and orientation of the tool center point can be controlled more precisely using external laser trackers than by the systems themselves, which are subject to load-dependent deformation. The model for the compensation of the kinematic errors uses the more precise position information of the laser trackers as well as the ambient temperature, the internal sensor system and the information on the axis errors (Schmitt et al., 2013b; Blunt, 2013; Altintas et al., 2005; Schwenke et al., 2005).
An example of the model-based and requirement-oriented usage of the measurement systems themselves has already been implemented for a distributed measurement system of infrared cameras (Franceschini et al., 2016, 2012; Galetto et al., 2015, 2010). The goal is to optimize the number of sensors needed in a sensor network. The measurement uncertainty depends on the number and the position of the sensors used and can be calculated for each configuration using the developed model. If a limit value for the measurement uncertainty exists, the service for determining the measured value can select and request an economically optimal number of sensors. The measurement uncertainty becomes the manipulated variable in the production control. In this way, the measurement uncertainty can automatically be optimized as a target value in the production scenario. It is thus possible, for example, to ensure that only the number of sensors is used that is actually required and thus the efficiency of the measurement is increased. Unnecessary sensors can be used for other measurement tasks. In contrast to the models for virtual measurement systems (see Sect. 3.3), this is an external model and not a measurement process-integrated model.
2.3 Need for service-based sensor information
As described in the sections before, the focus of development in metrology will shift from device engineering to the provision and model-based linking of data. The deployment, inter-connection and flexibility of production in the vision of cyber-physical production systems is a central field of development and opportunity for metrology. The key to the implementation of model-based process control is sensor systems, which allow for a holistic and valid recording of the current state of the production scenarios. The central requirement for measurement technology is therefore a flexible and demand-oriented provision of homogenized measurement data from distributed measurement systems. One possible solution to meet this requirement is the modularized, standardized and model-based provision of sensor information using the concept of sensor information as a service, which allows an application-oriented high specificity. This concept of sensor information as a service as well as its stages of development and application scenarios are presented in the following chapters.
The concept of sensor information as a service (S2aaS, see Kantarci and Mouftah, 2015; Perera et al., 2014b; Sheng et al., 2013; Zaslavsky et al., 2012) is based on a service-oriented architecture in computer science, which is increasingly applied to business processes with a high demand of flexibility and compatibility in distributed processes (Candido et al., 2011; Bell, 2012; Rosen, 2008). The core aspect is to divide business processes into individual activities and to encapsulate them with clearly defined tasks. The modules offer their functionalities in the form of services. The service consists of a retrievable service that is fixedly defined towards external users, without the implementation or execution of the service being visible. Communication is done via standardized interfaces. Due to the division into hierarchical levels and the access of higher services to services of lower hierarchical levels, complex services can be processed in a modularized manner. The two basic principles of a service-oriented architecture are the separation of responsibilities according to the provided services as well as the encapsulation of the technical details of the implementation. The goal is to maximize the reusability of the services and to maximize a flexible usage of the output. Sensor information as a service is therefore understood as the connection of physical sensors with (software) services, which can be accessed in a standardized manner using the Internet or local networks. The service provides one or more measurement or (relevant) status values as the primary output. However, for the user the generation and acquisition of the values stays hidden. Similar to the concept of everything as a service, the physical implementation and processing is separated from the provision of sensor data (Candido et al., 2010; Krafzig et al., 2005; Rosen, 2008). For the concept of sensor information as a service, it is therefore necessary in the first step to link physical sensors with services; see Fig. 4. Therefore the encapsulation levels for sensor systems must be expanded. Previously, sensors were designed without interfaces, but sensor information as a service requires interfaces to the Internet or local networks. By combining services of low abstraction levels (for example 1-D position information), services of higher abstraction levels (for example, 6-D position information) can be created. The scope of the services ranges from providing the hardware and the recording of the sensor data via the filtering and pre-processing of the data to the combination of several individual sensors.
Advantages of the service concept are generally a high degree of standardization, high flexibility, more availability and lower investment costs. With regard to metrology, this means that the two requirements of being more flexible and faster can be met by the sensor information as a service. The increase in flexibility is achieved by the fact that, if necessary, a large number of measured values are universally available and are initially offered without a direct application. In many cases, existing sensors can be used for tasks for which they were not intended in the first place. Therefore, services can be designed specifically for 1 : 1, n : 1, 1 : n or m : n links, but services can also access output information from other services. Their heterogeneity, combined with their specificity, make measurement data and feature values available more quickly by using the standardized and universal connectivity of the sensors as well as by using an automated analysis and combination of the sensor data. However, an increase in the accuracy or reduction of the measurement uncertainty in distributed systems can also be achieved by the service concept. If, for example, the measurement uncertainty is too high for the application scenario and a service supports the exchange or the addition of measurement systems, further sensors, possibly with another modality, can be addressed, which then in combination have a lower measurement uncertainty.
3.1 Implementation of the service concept
For the implementation, a service-oriented architecture of sensor data acquisition and processing is necessary. The first applicable approaches are described by Kantarci and Mouftah (2015), Perera et al. (2014b, c), Sheng et al. (2013) and Ibbotson et al. (2010). Zaslavsky et al. (2012), Broring et al. (2011) and Yuriyama and Kushida (2010) describe the connection of the sensors to the Internet and the cloud. On the one hand, a homogenization of the recorded data and of the communication interfaces is necessary for the concept of the sensor information as a service and, on the other hand, methods are necessary to validate the functionality of the services and to calibrate measurements carried out by the services. The homogenization of the interfaces is necessary, especially for the connection of arbitrary sensors and the establishment of service hierarchies as well as the universal use of these services for data analysis and within feedback loops. Homogenization involves the standardization of protocols, request structures, interfaces and data formats. In the future, the question will be how distributed data sets can be stored and described in the individualized production and how to deal with heterogeneous data. The goal is a flexible and product-centered collection of production process data, which is organized using partial homogenization and is provided ad hoc to users.
In order to acquire valid and comparable measurement data with a service, the systems for the generation and determination of the measurement and sensor information have to be calibrated. However, this requires, in addition to the calibration of the physical measurements, a calibration of the evaluation algorithms used in the service as well as a calibration of the underlying models – a change from the previous approaches. Although for a long time models have been used for the calculation of measurement uncertainties, e.g., according to the “Guide to the Expression of Uncertainty in Measurement” (GUM; the so-called measurement model), these are usually only validated experimentally. However, the models described above require a structural validation. Therefore, calibration methods are more and more required for the validity of the underlying logic and its implementation. These virtual standards serve as a reference for the calibration of the services. For software there are already approaches to solve this challenge using reference data sets, which are provided, for example, by central authorities such as the Physikalisch-Technische Bundesanstalt (PTB) or the International Organization for Standardization (ISO). Examples are the TraCIM project (PTB, 2017b), reference data sets for roughness measurements (PTB, 2017a) or reference data sets for the implementation of statistical process control (ISO, 2016). The additional software-specific traceability can also be used in the future in the case of more complex measurement systems, for example for computed tomography, in order to improve the traceability of the physical measurement in that the error of the classical calibration is already compensated beforehand by the error of the evaluation. In other words, the knowledge that is reflected in the application-specific digital shadow of the service modifies the underlying model itself.
The increasing number of sensors in use makes a regular and economically acceptable calibration using standards and references and the associated classic traceability more difficult. In order to be able to use these sensors for measurement tasks in the future and to ensure the validity of the data without regular calibration, current work focusses on fundamental self-calibration strategies and procedures to which the metrological infrastructure must then be adapted. Self-calibration has long been relevant to industries in which long-lasting sensors are used and which are not accessible to recalibration, e.g., in space travel or meteorology. Within these application scenarios, first methods for self-calibration can be found.
The methods themselves are based on different approaches. A common method is the use of substitute references. For example, the use of known quantities in the acquired image area for the calibration of optical systems (Ling and Strohmer, 2015; Lu et al., 2013), the calibration of inertial sensors via built-in microchips, which produce reference Coriolis forces (Aktakka and Najafi, 2016) or the usage of quantum-based electrical standards as developed by NIST; see for example the “NIST-on-a-Chip” program. (Flowers-Jacobs et al., 2016). A method specific to the area of multi-sensor systems is checking the consistency with other identical sensors and matching their calibration status (Goos et al., 2003; Kelly and Sukhatme, 2011; Dai et al., 2016). Other methods are based on internal consistency checks and mathematical methods for verifying validity, and can also be used, for example, for the calibration of coordinate measurement devices (Dang et al., 2006; van der Horn and Huijsing, 2011). As described in Sect. 2, models for controlling real production processes will be modular in the future. The data required in the models for control are then requested from services instead of directly from sensors and are provided with all necessary additional information (see Fig. 4). The development effort for new models is divided into the development of service-internal models and the modeling of the production processes. The development of the models for the services includes, on the one hand, models of the sensors and, on the other, models for combining sensors with higher order services. For example, a service that provides a pose, but which must combine this pose from several different sub-services using different measurement systems (laser tracker, iGPS, robot control), requires a model for the acquisition, combination and validation of the individual measurement systems. Generally speaking, with the use of services that use heuristics and adaptive learning methods, sensor data transform into smart data, which enables manual or automated event-driven decisions and adaptive behavior.
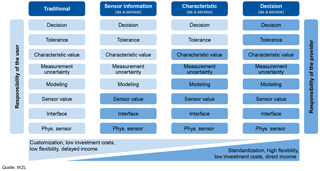
Figure 5Development stages of the service concept in metrology, translated from Schmitt and Voigtmann (2017).
Another focus of development will be on the methods for a measurement system analysis and process capability studies as well as on the methods for process monitoring as these methods have to be adapted for the use on and with services. In particular, the process monitoring will shift from the monitoring of individual sensors to the monitoring of services and virtual sensor groups.
3.2 Development stages of the service concept
Analogous to the development of the concept of software as a service to the concept of Platform as a Service, the development of the sensor information as a service can be taken further, so that the services will take on additional tasks. Figure 5 shows, based on the development stages of the concept software as a service, different levels of services for metrology and different stages of outsourcing of individual tasks as a service. The responsibility and the management of the tasks shift from left to right more and more to the service provider. The first step is that without the provision of a measurement uncertainty, the value of a characteristic provided by a service is also worthless. For the determination of the measurement uncertainty, a large amount of information about the environmental conditions, as well as models for linking this information to the measurand, is necessary. Therefore, for the concept of “characteristic as a service” in addition to the value of the characteristic, all environmental conditions of the process and all uncertainties must be determined and combined. This information itself can, in turn, be collected using services or must be provided by the services for the sensor information in addition to the sensor measurement value. This means, that the provision of meta-information (temperatures, ambient conditions, calibration state, time and time dependency, location, etc.) must be included in the services, and mere provision of the sensor and measured values is not sufficient. Only with knowledge of the meta-information can a complete measurement uncertainty be derived via previously created models (measurement model). The starting point for the second step is the consideration that the relevant added value when using measurement data does not lie in the measurement data themselves or their analysis, but only in the subsequent decision, e.g., the decision to intervene to achieve process optimization or the decision for or against product conformity. From a user perspective, only this decision must be made available within an approved error probability. It is not important which sensors are used, how characteristics or process data are extracted or how large the tolerances for process control or product conformity are – for the user it is only important whether the value is within the tolerance limits and with what error probability this decision can be made. The uncertainty of the decision is determined using the ratio of the uncertainty of the determination of the measured values in the application and the distance to the tolerance limit. The tolerance itself can also be provided using a service, which may perform adaptive and dynamic tolerance adjustments. “Decision as a service” thus forms the highest hierarchy level and uses subordinate services, such as the provision of tolerance or the provision of the characteristic value.
Consequently, the provision of the physical sensor itself can also be offered as a service. In the cyber-physical production systems, described in Sect. 2.1, in which measurement systems are also mobile (e.g., employees' smartphones, laser trackers mounted on drones, CT devices on mobile platforms), the demand-dependent (e.g., measurement uncertainty-dependent or frequency-dependent) measurement systems can be automatically transported to the required location using services.
3.3 Application scenario: the virtual metrology room
A noticeable trend in metrology is the time shift between the direct recording of the characteristic on the product to a measurement of the characteristic on a model of the product. This means that at first a digital model of the product is acquired independently of the measurement task and stored digitally. Individual characteristics from this model can be retrospectively extracted, measured and evaluated. The product models consist not only of point clouds representing the 3-D geometry, but they also consist of models that include the environmental conditions, e.g., to compensate for deformation of products under the effect of force (Forbes, 2015; Maropoulos et al., 2008) or to compensate for thermal effects (Schmitt and Peterek, 2015). The digital product model therefore is more than just the digital representation of the component. By taking the ambient conditions into account, the model-based measurements can be used more precisely and more flexibly than the direct measurement of the characteristic. In semiconductor manufacturing, for example, virtual measurement processes are already used because of the large number of pieces and the impossibility of direct measurements (Khan et al., 2007). All available sensor information is used and combined in the digital shadow of the product to precisely represent the product geometry and the behavior of the product. The complete 3-D digitization of products using photogrammetry is a classic example of a purely digital shadow. An example of a model-based measurement method is the optimization of laser interferometry measurements. The additional measurement of air temperature, atmospheric humidity and atmospheric pressure in several locations can be used to calculate the refractive index in the entire working area with the help of spatial statistics, thus improving the conversion of optical lengths to geometric lengths and thus the interferometry measurements. The model is flexible in such a way that acoustic tomography can also be used for the indication of the ambient temperature referenced by location and time (Schmitt et al., 2016).
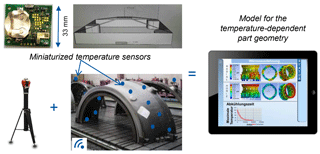
Figure 6Example of a virtual measurement process: compensation of geometry errors via temperature models of the component within the production environment. This figure is translated from Schmitt and Voigtmann (2017).
Another trend to reduce the uncertainty of the measurements and to increase the flexibility is virtual measurement processes. For the automated determination of value of a characteristic and its measurement uncertainty, it is necessary to automatically determine the measurement uncertainty under application conditions. For this purpose, application-specific models of the measurement processes for combining and simulating the influences on the measurement and the associated uncertainties are necessary. This is called a virtual measurement process. Typical examples are the virtual coordinate measurement machine or the virtual laser tracker. In both cases, the measurement uncertainty of a real-world measurement is estimated by simulation using environmental data as well as a model of the influence of the environmental conditions on the measurement uncertainty (Schmitt et al., 2016; Huo et al., 2009, 2010; Trapet, 1999). Virtual measurement processes form the basis for a future a priori assessment of the measurement uncertainty and thus the basis for an optimization of the measurement planning. With virtual measurement processes, there is also a chance that air-conditioned measurement rooms are no longer necessary to measure at reference temperatures but that they can be replaced by a sensor-assisted temperature recording and models for the temperature behavior of the measurement processes and the products within the production environment. A contribution to the compensation of temperature-related geometry errors in the production can be found in Ohlenforst et al. (2016, see Fig. 6). The integration of miniaturized temperature sensors into the product and the exact measurement of their position using laser trackers as well as a complete model of the product enable the compensation of temperature-related geometry errors. If these models are extended from the temperature to all ambient influences, which are kept constant in measurement rooms, it is foreseeable that, especially in production processes of technologically or dimensionally complex products, classical production approaches with a dedicated measurement space will be replaced or supplemented by virtual measurement processes in combination with the recording of the environmental data in the production. If the structure of the virtual measurement processes and the inputted production data are developed as services, they can react flexibly to different products and production conditions. A virtual metrology room is created according to current needs, which, if necessary, can be reconfigured locally and temporally in the production.
3.4 Stakeholders
The service administrators and cloud service providers will be affected by this development on the one hand. For them, the effort to implement and maintain the services will increase. On the other hand, the users of the services will be able to simplify their acquisition of measurement data. A strong separation is taking place between experts of metrology and users of metrology. For users, the complexity of the information generation is reduced by the provision of simplified operating and adjustment concepts in favor of their competence to act as smart experts in their respective domains, e.g., the production. For sensor manufacturers, this means, in particular, a stronger focus on intelligent sensors, which are also referred to as smart sensors or sensor 4.0 (Schütze and Helwig, 2017; Rice, 2009; Meijer, 2008; Spencer et al., 2004), and their communication interfaces as well as their compatibility to standard data formats. However, it is unclear who will be the driving force behind this development and provision of these services. Whether this development is more likely to originate from sensor manufacturers, production-related software producers or non-production-related software providers (e.g., providers of cloud services or big data analysis) remains to be seen. It is possible that the large providers of cloud services and search engines, with their already existing know-how in the field of service-oriented concepts, will expand their business models.
The conversion to a service-based application of metrology also makes it possible to re-evaluate the cost-effectiveness of metrology. One of the main problems of metrology results from accounting: metrology components are often large investments, and rooms and personnel are billed as overhead costs; an estimate of their benefit in the sense of a contribution to the efficiency of the production is often impossible. Typically, services are billed per usage or for a period of use. Eventually, when services are offered not only internally, but also between companies, the value of the services, and thus the value of metrology, must be stated per usage or for a period of use. Depending on the scope of the service, this requires the determination of how much the value of sensor information, characteristic values, measurement uncertainties or decisions is per indication or per usage and how this is quantified. If this is the case, the value of the metrology or the value of the data provided and thus the usefulness of metrology can be presented more obviously. The central questions for the evaluation of sensor information as a service are as follows. What does sensor information cost? What does an inspection cost? What is the benefit for metrology per application? Sensor-related services here also allow for a better integration of metrology into business processes.
The digitization, networking and improvement in flexibility of production means, which lead to cyber-physical production systems and which can flexibly adapt to customer requirements, are central developments in production and an opportunity for metrology. In networked production, metrology forms the basis for a holistic, valid assessment of production scenarios and is the key for the implementation of a model-based process control. As a result, the development focus of metrology will shift from device engineering to the provision and linking of data. The main challenges for networking are the homogenization of data formats and interfaces. With the concept of sensor information as a service, a high degree of standardization and modularization can be achieved, which makes it possible to use sensors and measurement systems more flexibly and in a more appropriate manner. Therefore, it can be used as a central component for the creation of future cyber-physical production systems. Approaches and initial solutions for a service-oriented architecture of sensor systems and the connection to cloud solutions are already available, but further research and development is necessary for a comprehensive integration into production.
No data sets were used in this article.
The authors declare that they have no conflict of interest.
This article is part of the special issue “Evaluating measurement data and uncertainty”. It does not belong to a conference.
This is an updated and revised version of a paper written in German language
and published in “Technisches Messen 84 (2017) 2” in 2017. It is published
in JSSS with the kind permission of the publisher De Gruyter.
Edited by: Klaus-Dieter Sommer
Reviewed by:three anonymous referees
Aktakka, E. E. and Najafi, K.: A six-axis micro platform for in situ calibration of MEMS inertial sensors, in: 2016 IEEE 29th International Conference on Micro Electro Mechanical Systems (MEMS), 24–28 January 2016, Shanghai, China, 243–246, 2016.
Altintas, Y., Brecher, C., Weck, M., and Witt, S.: Virtual Machine Tool, CIRP Ann.-Manuf. Techn., 54, 115–138, https://doi.org/10.1016/S0007-8506(07)60022-5, 2005.
Barnaghi, P., Meissner, S., Presser, M., and Moessner, K.: Sense and Sens'ability: Semantic Data Modelling for Sensor Networks, ICT-MobileSummit 2009 Conference Proceedings, 10–12 June 2009, Santander, Spain, 2009.
Bauernhansl, T., Krüger, J., Reinhart, G., and Schuh, G.: Wgp-Standpunkt Industrie 4.0, available at: https://wgp.de/wp-content/uploads/WGP-Standpunkt_Industrie_4-0.pdf, last access: 12 January 2017.
Bell, M.: Service-Oriented Modeling, John Wiley & Sons, Inc, Hoboken, NJ, USA, 2012.
Blunt, L. (Ed.): Laser metrology and machine performance X: 10th International Conference and Exhibition on Laser Metrology, Machine Tool, CMM & Robotic Performance, Lamdamap 2013, 20–21 March 2013, Chicheley Hall, Newton Pagnell, Bucks, Buckinghamshire, UK, Euspen, Cranfield, 381 pp., 2013.
Brecher, C., Klocke, F., Schmitt, R., and Schuh, G. (Eds.): Integrative Produktion: Industrie 4.0 – Aachener Perspektiven, AWK, Aachener Werkzeugmaschinen-Kolloquium 2014, 22–23 May 2014, Tagungsband, Shaker, Aachen, Germany, 449 pp., 2014.
Brecher, C., Klocke, F., Schmitt, R., and Schuh, G. (Eds.): Internet of Production für agile Unternehmen: AWK Aachener Werkzeugmaschinen-Kolloquium 2017, 18–19 May 2017, Apprimus Verlag, Aachen, Germany, 460 pp., 2017.
Broring, A., Echterhoff, J., Jirka, S., Simonis, I., Everding, T., Stasch, C., Liang, S., and Lemmens, R.: New generation Sensor Web Enablement, Sensors (Basel, Switzerland), 11, 2652–2699, https://doi.org/10.3390/s110302652, 2011.
Broy, M.: Cyber-Physical Systems: Innovation Durch Software-Intensive Eingebettete Systeme, acatech DISKUTIERT, 0, Springer-Verlag Berlin Heidelberg, Berlin, Heidelberg, Germany, 2010.
Candido, G., Jammes, F., de Oliveira, J. B., and Colombo, A. W.: SOA at device level in the industrial domain: Assessment of OPC UA and DPWS specifications, in: 2010 8th IEEE International Conference on Industrial Informatics (INDIN), 13–16 July 2010, Osaka, Japan, 598–603, 2010.
Candido, G., Colombo, A. W., Barata, J., and Jammes, F.: Service-Oriented Infrastructure to Support the Deployment of Evolvable Production Systems, IEEE T. Ind. Inform., 7, 759–767, https://doi.org/10.1109/TII.2011.2166779, 2011.
Compton, M., Henson, C., Lefort, L., Neuhaus, H., and Sheth, A. (Eds.): A Survey of the Semantic Specification of Sensors, CEUR Workshop Proceedings, 21 April 2009, Madrid, Spain, 2009.
Dai, P., Wang, X., Tan, Y., Zhang, P., and Li, X.: Adaptive recursive optimized extrinsic self-calibration in distributed visual sensor networks, in: 2016 IEEE International Instrumentation and Measurement Technology Conference (I2MTC), 23–26 May 2016, Taipei, Taiwan, 1–6, 2016.
Dang, Q. C., Yoo, S., and Kim, S.-W.: Complete 3-D Self-calibration of Coordinate Measuring Machines, CIRP Ann.-Manuf. Techn., 55, 527–530, https://doi.org/10.1016/S0007-8506(07)60474-0, 2006.
DIN: Referenzarchitekturmodell Industrie 4.0 (RAMI4.0), Beuth, Berlin, Germany, 2016.
Duan, Y.: Value Modeling and Calculation for Everything as a Service (XaaS) Based on Reuse, in: 2012 13th ACIS International Conference on Software Engineering, Artificial Intelligence, Networking and Parallel & Distributed Computing (SNPD): Value Modeling and Calculation for Everything as a Service (XaaS) Based on Reuse, 8–10 August 2012, Kyoto, Japan, 162–167, 2012.
Duan, Y., Feng, W., Zhou, X., Dong, L., Hu, T., and Gao, H.: Exploring the Categories and Models of Everything as a Service (XaaS), IJGDC, 8, 215–228, https://doi.org/10.14257/ijgdc.2015.8.6.21, 2015.
Fenn, J. and Linden, A.: Gartner's hype cycle special report for 2005, available at: http://www.gartner.com/DisplayDocument?doc_cd=130115, last access: 12 January 2017.
Flowers-Jacobs, N. E., Fox, A. E., Dresselhaus, P. D., Schwall, R. E., and Benz, S. P.: Two-Volt Josephson Arbitrary Waveform Synthesizer Using Wilkinson Dividers, IEEE T. Appl. Supercon., 26, 1400207, https://doi.org/10.1109/TASC.2016.2532798, 2016.
Forbes, A.: Reference Models and Algorithms for Multi-Station Coordinate Metrology, XXI IMEKO World Congress ”Measurement in Research and Industry”, 30 August–4 September 2015, Prague, Czech Republic, 2015.
Forbes, A., Schmitt, R., Jatzkowski, P., and Quinders, S.: Metrology Infrastructure for Multi-Robotic Cooperative Assembly, in: ISMTII 2013: 11th International Symposium on Measurement Technology and Intelligent Instruments; Metrology, Master global challenges, 1–5 July 2013, RWTH, Aachen, Germany, 1st edn., edited by: Schmitt, R., Apprimus-Verl., Aachen, Germany, 54–62, 2013.
Franceschini, F., Galetto, M., Maisano, D., and Mastrogiacomo, L.: Uncertainty Model for Systems Based on Wireless Sensor Networks for Large Scale Dimensional Metrology, in: ASME 2012 11th Biennial Conference on Engineering Systems Design and Analysis, 2 July 2012, Nantes, France, 429–435, 2012.
Franceschini, F., Galetto, M., Maisano, D., and Mastrogiacomo, L.: Combining multiple Large Volume Metrology systems: Competitive versus cooperative data fusion, Precis. Eng., 43, 514–524, https://doi.org/10.1016/j.precisioneng.2015.09.014, 2016.
Galetto, M., Mastrogiacomo, L., and Pralio, B.: A wireless sensor network-based approach to large-scale dimensional metrology, Int. J. Comp. Integ. M., 23, 1082–1094, https://doi.org/10.1080/0951192X.2010.518322, 2010.
Galetto, M., Mastrogiacomo, L., Maisano, D., and Franceschini, F.: Cooperative fusion of distributed multi-sensor LVM (Large Volume Metrology) systems, CIRP Ann.-Manuf. Techn., 64, 483–486, https://doi.org/10.1016/j.cirp.2015.04.003, 2015.
Ghidary, S. S., Tani, T., Takamori, T., and Hattori, M.: A new home robot positioning system (HRPS) using IR switched multi ultrasonic sensors – Systems, Man, and Cybernetics, 1999. IEEE SMC '99 Conference Proceedings. 1999 IEEE International, Proc. IEEE SMC99, IV-737–IV-741, 1999.
Goos, G., Hartmanis, J., van Leeuwen, J., Zhao, F., and Guibas, L. (Eds.): Information Processing in Sensor Networks, Lecture notes in computer science, Springer Berlin Heidelberg, Berlin, Heidelberg, Germany, 2003.
Guenther, R. and Radebaugh, J.: Understanding metadata, NISO Press, Bethesda, MD, USA, 16 pp., 2004.
Hannelius, T., Salmenpera, M., and Kuikka, S.: Roadmap to adopting OPC UA, in: 6th IEEE International Conference on Industrial Informatics (INDIN), 13–16 July 2008, Daejeon, South Korea, 756–761, 2008.
Huang, V. and Javed, M. K. (Eds.): Semantic Sensor Information Description and Processing, IEEE Computer Society, Piscataway, NJ, USA, 1 p., 2008.
Huettemann, G., Gaffry, C., and Schmitt, R. H.: Adaptation of Reconfigurable Manufacturing Systems for Industrial Assembly – Review of Flexibility Paradigms, Concepts, and Outlook, Proc. CIRP, 52, 112–117, https://doi.org/10.1016/j.procir.2016.07.021, 2016.
Huo, D., Marapoulos, P. G., and Cheng, C. H.: The framework of the virtual laser tracker – a systematic approach to the assessment of error sources and uncertainty in laser tracker measurement, in: 6th CIRP-Sponsored International Conference on Digital Enterprise Technology, 14–16 December 2009, Hong Kong, 507–523, 2009.
Huo, D., Maropoulos, P. G., and Cheng, C.H.: The Framework of the Virtual Laser Tracker – A Systematic Approach to the Assessment of Error sources and Uncertainty in Laser Tracker Measurement, in: Proceedings of the 6th CIRP-Sponsored International Conference on Digital Enterprise Technology. Advances in Intelligent and Soft Computing, edited by: Huang, G. Q., Mak, K. L., and Maropoulos, P. G., Springer, Berlin, Heidelberg, vol. 66, 507–523, https://doi.org/10.1007/978-3-642-10430-5_39, 2010.
Ibbotson, J., Gibson, C., Wright, J., Waggett, P., Zerfos, P., Szymanski, B., and Thornley, D. J.: Sensors as a Service Oriented Architecture: Middleware for Sensor Networks, in: 2010 6th International Conference on Intelligent Environments (IE), 19–21 July 2010, Kuala Lumpur, Malaysia, 209–214, 2010.
Imkamp, D., Schmitt, R., and Berthold, J.: Blick in die Zukunft der Fertigungsmesstechnik, tm – Technisches Messen, 79, 433–439, https://doi.org/10.1524/teme.2012.0251, 2012.
Imkamp, D., Berthold, J., Heizmann, M., Kniel, K., Peterek, M., Schmitt, R., Seidler, J., and Sommer, K.-D.: Herausforderungen und Trends in der Fertigungsmesstechnik – Industrie 4.0, tm – Technisches Messen, 83, 417–429, https://doi.org/10.1515/teme-2015-0081, 2016.
ISO: Guidelines for implementation of statistical process control (SPC) – Part 3: SPC on test examples for software validation (ISO TR 11462-3), available at: http://www.iso.org/iso/home/store/catalogue_tc/catalogue_detail.htm?csnumber=69644 (last access: 18 November 2017), 2016.
ISO: Industrial automation systems and integration – Product data representation and exchange (ISO 10303 series), Beuth, Berlin, Germany, 2018.
JCGM: Evaluation of measurement data – Guide to the expression of uncertainty in measurement (JCGM 100:2008), available at: http://www.bipm.org/utils/common/documents/jcgm/JCGM_100_2008_E.pdf (last access: 15 January 2018), 2008.
Kantarci, B. and Mouftah, H. T.: Sensing as a Service in Cloud-Centric Internet of Things Architecture, in: Enabling Real-Time Mobile Cloud Computing through Emerging Technologies, 1st edn., edited by: Soyata, T., IGI Global, University of Rochester, USA, 83–115, 2015.
Kelly, J. and Sukhatme, G. S.: Visual-Inertial Sensor Fusion: Localization, Mapping and Sensor-to-Sensor Self-calibration, Int. J. Robot. Res., 30, 56–79, https://doi.org/10.1177/0278364910382802, 2011.
Khan, A. A., Moyne, J. R., and Tilbury, D. M.: An Approach for Factory-Wide Control Utilizing Virtual Metrology, IEEE T. Semiconduct. M., 20, 364–375, https://doi.org/10.1109/TSM.2007.907609, 2007.
Kim, J.-H., Kwon, H., Kim, D.-H., Kwak, H.-Y., and Lee, S.-J.: Building a Service-Oriented Ontology for Wireless Sensor Networks, in: Seventh IEEE/ACIS International Conference on Computer and Information Science (icis 2008), 14–16 May 2008, Portland, Oregon, USA, 649–654, 2008.
Klocke, F., Lung, D., and Puls, H.: FEM-Modelling of the Thermal Workpiece Deformation in Dry Turning, Proc. CIRP, 8, 240–245, https://doi.org/10.1016/j.procir.2013.06.096, 2013.
Koza, J. R.: Genetic programming: automatic synthesis of topologies and numerical parameters, Handbook of Metaheuristics, 83–104, 2003.
Krafzig, D., Banke, K., and Slama, D.: Enterprise SOA: Service-oriented architecture best practices, The Coad series, Prentice Hall Professional Technical Reference, Indianapolis, IN, USA, xxvi, 418 pp., 2005.
Leach, R.: Information-rich metrology: a fresh approach to manufacturing metrology, 66th CIRP General Assembly, 21–27 August 2016, Guimaraes, Portugal, https://doi.org/10.13140/RG.2.2.34821.76005, 2016.
Li, S., Xu, L. D., and Wang, X.: Compressed Sensing Signal and Data Acquisition in Wireless Sensor Networks and Internet of Things, IEEE T. Ind. Inform., 9, 2177–2186, https://doi.org/10.1109/TII.2012.2189222, 2013.
Ling, S. and Strohmer, T.: Self-calibration and biconvex compressive sensing, Inverse Probl., 31, 115002, https://doi.org/10.1088/0266-5611/31/11/115002, 2015.
Löwen, U., Braune, A., Diesner, M., Hüttemann, G., Klein, M., Thron, M., Manger, T., and Okon, M.: Industrie 4.0 Components – Modeling Examples, VDI Technical Report, Berlin, Germany, https://doi.org/10.13140/RG.2.2.29092.68488, 2016.
Löwen, U., Braune, A., Diesner, M., Hüttemann, G., Klein, M., Thron, M., Manger, T., Okon, M., and Kochseder, R.: Exemplification of the Industrie 4.0 Application Scenario Value-Based Service following IIRA Structure, Federal Ministry for Economic Affairs and Energy (BMWi), Berlin, Germany, 2017.
Lu, J., Gong, J., Hao, Q., and Hu, F.: Multi-agent based wireless pyroelectric infrared sensor networks for multi-human tracking and self-calibration, in: 2013 IEEE Sensors, Baltimore, MD, USA, 1–4, 2013.
Maropoulos, P. G., Guo, Y., Jamshidi, J., and Cai, B.: Large volume metrology process models: A framework for integrating measurement with assembly planning, CIRP Ann.-Manuf. Techn., 57, 477–480, https://doi.org/10.1016/j.cirp.2008.03.017, 2008.
Maropoulos, P. G., Vichare, P., Martin, O., Muelaner, J., Summers, M. D., and Kayani, A.: Early design verification of complex assembly variability using a Hybrid – Model Based and Physical Testing – Methodology, CIRP Ann.-Manuf. Techn., 60, 207–210, https://doi.org/10.1016/j.cirp.2011.03.097, 2011.
Meijer, G. C. M.: Smart sensor systems, 1 online resource, J. Wiley & Sons, Chichester, UK, xvii, 404 pp., 2008.
Möhring, H.-C., Litwinski, K. M., and Gümmer, O.: Process monitoring with sensory machine tool components, CIRP Ann.-Manuf. Techn., 59, 383–386, https://doi.org/10.1016/j.cirp.2010.03.087, 2010.
Monostori, L.: Cyber-physical Production Systems: Roots, Expectations and R&D Challenges, Proc. CIRP, 17, 9–13, https://doi.org/10.1016/j.procir.2014.03.115, 2014.
Monostori, L., Kádár, B., Bauernhansl, T., Kondoh, S., Kumara, S., Reinhart, G., Sauer, O., Schuh, G., Sihn, W., and Ueda, K.: Cyber-physical systems in manufacturing, CIRP Annals, 65, 621–641, https://doi.org/10.1016/j.cirp.2016.06.005, 2016.
Montavon, B., Peterek, M., and Schmitt, R. H.: Communication architecture for multiple distributed large volume metrology systems, in: 2017 IEEE International Systems Engineering Symposium (ISSE), 11–13 October 2017, Vienna, Austria, 1–5, 2017.
NetCDF 2017: Network Common Data Form, available at: http://www.unidata.ucar.edu/software/netcdf/, last access: 12 January 2017.
Ohlenforst, M., Dahlem, P., Peterek, M., and Schmitt, R.: Geometriemessungen auf Werkzeugmaschinen: Vorgehen zum Bestimmen und Minimieren der Messunsicherheit, Werkstatttechnik online, 106, 782–786, 2016.
O'Leary, D. E.: The Impact of Gartner's Maturity Curve, Adoption Curve, Strategic Technologies on Information Systems Research, with Applications to Artificial Intelligence, ERP, BPM, and RFID, Journal of Emerging Technologies in Accounting, 6, 45–66, https://doi.org/10.2308/jeta.2009.6.1.45, 2009.
Pabst, R., Fleischer, J., and Michna, J.: Modelling of the heat input for face-milling processes, CIRP Ann.-Manuf. Techn., 59, 121–124, https://doi.org/10.1016/j.cirp.2010.03.062, 2010.
Perera, C., Zaslavsky, A., Christen, P., and Georgakopoulos, D.: Context Aware Computing for The Internet of Things: A Survey, IEEE Commun. Surv. Tut., 16, 414–454, https://doi.org/10.1109/SURV.2013.042313.00197, 2014a.
Perera, C., Zaslavsky, A., Christen, P., and Georgakopoulos, D.: Sensing as a service model for smart cities supported by Internet of Things, Trans. Emerging Tel. Tech., 25, 81–93, https://doi.org/10.1002/ett.2704, 2014b.
Perera, C., Zaslavsky, A., Liu, C. H., Compton, M., Christen, P., and Georgakopoulos, D.: Sensor Search Techniques for Sensing as a Service Architecture for the Internet of Things, IEEE Sensors J., 14, 406–420, https://doi.org/10.1109/JSEN.2013.2282292, 2014c.
Permin, E., Bertelsmeier, F., Blum, M., Bützler, J., Haag, S., Kuz, S., Özdemir, D., Stemmler, S., Thombansen, U., Schmitt, R., Brecher, C., Schlick, C., Abel, D., Poprawe, R., Loosen, P., Schulz, W., and Schuh, G.: Self-optimizing Production Systems, Proc. CIRP, 41, 417–422, https://doi.org/10.1016/j.procir.2015.12.114, 2016.
PTB: Referenzsoftware für die Rauheitsmesstechnik, available at: http://www.ptb.de/cms/ptb/fachabteilungen/abt5/fb-51/ag-515/referenzsoftware515.html, last access: 12 January 2017a.
PTB: Traceability for Computationally-Intensive Metrology (TraCIM), available at: http://www.ptb.de/emrp/tcim.html, last access: 12 January 2017b.
Qingping, C., Hairong, Y., Chuan, Z., Zhibo, P., and Li, D. X.: A Reconfigurable Smart Sensor Interface for Industrial WSN in IoT Environment, IEEE T. Ind. Inform., 10, 1417–1425, https://doi.org/10.1109/TII.2014.2306798, 2014.
Rajkumar, R., Lee, I., Sha, L., and Stankovic, J.: Cyber-physical systems, in: the 47th Design Automation Conference, edited by: Sapatnekar, S., 13—18 June 2010, Anaheim, California, USA, 731–736, 2010.
Rice, J. A.: Flexible smart sensor framework for autonomous full-scale structural health monitoring, PhD Thesis, University of Illinois, Urbana-Champaign, USA, 303 pp., 2009.
Rosen, M.: Applied SOA: Service-Oriented Architecture and design strategies, Wiley, Indianapolis, Ind, USA, 2008.
Schmitt, R. and Peterek, M.: Traceable Measurements on Machine Tools – Thermal Influences on Machine Tool Structure and Measurement Uncertainty, Proc. CIRP, 33, 576–580, https://doi.org/10.1016/j.procir.2015.06.087, 2015.
Schmitt, R., Monostori, L., Glöckner, H., and Viharos, Z. J.: Design and assessment of quality control loops for stable business processes, CIRP Ann.-Manuf. Techn., 61, 439–444, https://doi.org/10.1016/j.cirp.2012.03.055, 2012.
Schmitt, R., Jatzkowski, P., Janssen, M., and Bertelsmeier, F.: Self-optimization in Large Scale Assembly, Procedia Engineer., 63, 843–851, https://doi.org/10.1016/j.proeng.2013.08.236, 2013a.
Schmitt, R., Jatzkowski, P., and Peterek, M.: Traceable measurements using machine tools, in: Laser metrology and machine performance X: 10th International Conference and Exhibition on Laser Metrology, Machine Tool, CMM & Robotic Performance, Lamdamap 2013, 20–21 March 2013, Chicheley Hall, Newton Pagnell, Bucks, Buckinghamshire, UK, edited by: Blunt, L., Euspen, Cranfield, UK, 133–143, 2013b.
Schmitt, R., Peterek, M., and Quinders, S.: Concept of a virtual metrology frame based on absolute interferometry for multi robotic assembly: 7th IFIP WG 5.5 International Precision Assembly Seminar, IPAS 2014, 16–18 February 2014, Chamonix, France, Revised Selected Papers, IFIP Advances In Information And Communication Technology, 79–86, 2014.
Schmitt, R. H. and Voigtmann, C.: Sensorinformationen als Dienst – Baustein der vernetzten Produktion, tm – Technisches Messen, 84, 296–309, https://doi.org/10.1515/teme-2017-0006, 2017.
Schmitt, R. H., Peterek, M., Morse, E., Knapp, W., Galetto, M., Härtig, F., Goch, G., Hughes, B., Forbes, A., and Estler, W. T.: Advances in Large-Scale Metrology – Review and future trends, CIRP Ann.-Manuf. Techn., 65, 643–665, https://doi.org/10.1016/j.cirp.2016.05.002, 2016.
Schuh, G., Anderl, R., Gausemeier, J., ten Hompel, M., and Wahlster, W. (Eds.): Industrie 4.0 Maturity Index: Die digitale Transformation von Unternehmen gestalten, acatech STUDIE, Herbert Utz Verlag, München, Germany, 55 pp., 2017.
Schütze, A. and Helwig, N.: Sensorik und Messtechnik für die Industrie 4.0, tm – Technisches Messen, 84, 310–319, https://doi.org/10.1515/teme-2016-0047, 2017.
Schwenke, H., Franke, M., Hannaford, J., and Kunzmann, H.: Error mapping of CMMs and machine tools by a single tracking interferometer, CIRP Ann.-Manuf. Techn., 54, 475–478, https://doi.org/10.1016/S0007-8506(07)60148-6, 2005.
Sheng, X., Tang, J., Xiao, X., and Xue, G.: Sensing as a Service: Challenges, Solutions and Future Directions, IEEE Sensors J., 13, 3733–3741, https://doi.org/10.1109/JSEN.2013.2262677, 2013.
Soyata, T.: Enabling Real-Time Mobile Cloud Computing through Emerging Technologies, IGI Global, 2015, 1–399, https://doi.org/10.4018/978-1-4666-8662-5, 2015.
Spencer, B. F., Ruiz-Sandoval, M. E., and Kurata, N.: Smart sensing technology: opportunities and challenges, Struct. Control Hlth., 2004, 349–368, 2004.
Trapet, E.: Traceability of coordinate measurements according to the method of the virtual measuring machine: Part 2 of the final report project MAT1-CT94-0076, PTB-Bericht F, 35, Wirtschaftsverl. NW, Bremerhaven, Germany, 147 pp., 1999.
van der Horn, G. and Huijsing, J. H.: Integrated smart sensors: Design and calibration, The Springer international series in engineering and computer science, vol. 419, 202 pp., Springer, New York, USA, London, UK, 2011.
VDI: Status Report Reference Architecture Model, available at: https://www.vdi.de/fileadmin/vdi_de/redakteur_dateien/gma_dateien/5305_Publikation_GMA_Status_Report_ZVEI_Reference_Architecture_Model.pdf, last access: 12 January 2017.
Wang, Z., Liang, M., and Maropoulos, P. G.: High accuracy mobile robot positioning using external large volume metrology instruments, Int. J. Comp. Integ. M., 24, 484–492, https://doi.org/10.1080/0951192x.2011.554868, 2011.
World Economic Forum: Digital Transformation of Industries: Consumer Industries, available at: http://reports.weforum.org/digital-transformation-of-industries/, last access: 12 January 2017.
Wu, D., Greer, M. J., Rosen, D. W., and Schaefer, D.: Cloud manufacturing: Strategic vision and state-of-the-art, J. Manuf. Syst., 32, 564–579, https://doi.org/10.1016/j.jmsy.2013.04.008, 2013.
Xu, X.: From cloud computing to cloud manufacturing, Robot. Cim.-Int. Manuf., 28, 75–86, https://doi.org/10.1016/j.rcim.2011.07.002, 2012.
Yang, H. and Tate, M.: A Descriptive Literature Review and Classification of Cloud Computing Research, in: Communications of the Association 2012, vol. 31, 35–60, available at: http://aisel.aisnet.org/cais/ (last access: 1 March 2018), 2012.
Yuriyama, M. and Kushida, T. (Eds.): Sensor-Cloud Infrastructure: Physical Sensor Management with Virtualized Sensors on Cloud Computing, IEEE 13th International Conference on Network-Based Information Systems, 14–16 September 2010, Takayama, Japan, https://doi.org/10.1109/NBiS.2010.32, 2010.
Zaslavsky, A., Perera, C., and Georgakopoulos, D. (Eds.): Sensing as Service and Big Data: Advances in cloud computing, Univ. Press, Hyderabad, India, 165 pp., 2012.
sensor information as a serviceis presented as a key development for the flexible use of metrology.