the Creative Commons Attribution 4.0 License.
the Creative Commons Attribution 4.0 License.
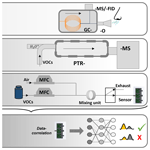
A concept for sensor system developments using raw-milk monitoring as a case study
Maximilian Koehne
Michael Henfling
Kristina Amtmann
Andreas Stenzel
Andrea Buettner
Sabine Trupp
Tilman Sauerwald
In this work, we present a concept for a raw-milk monitoring sensor system aiming at demonstrating a generalized approach for low-cost gas sensor system development in future. These systems are expected to be comparatively less expensive than conventional gas chromatography (GC) systems and can therefore likewise be used by farmers to monitor on-site storage as well as by dairy companies for the inspection of incoming milk and can thus play a significant role in counteracting the waste of milk and its products. This generalizable method is based on three steps: identification of potential milk degradation markers, quantification of these markers, and characterization of metal oxide semiconductor (MOS) sensors for these markers. In the first step, gas chromatography–mass spectrometry (GC-MS) and GC–flame ionization detector (GC-FID)/olfactometry (O) were used to tentatively identify 14 volatile substances in the headspace concentrations above the raw milk. From this, 3-methylbutan-1-ol, hexan-1-ol, pentan-1-ol, acetic acid, and additionally ethanol and ethyl acetate were selected by cross-referencing our results with literature data. In addition, hexanal, 2-methyl-1-propanol, limonene, nonanal, 2-ethylhexan-1-ol, butanoic acid, hexanoic acid, octanoic acid, methyl hexadecanoate, and decanoic acid were identified but not selected as potential markers due to their properties being incompatible with gas mixing apparatus (GMA). In the second step, a proton transfer reaction–MS (PTR-MS) analysis was used to determine the concentration in the headspace, which is in the parts per billion (ppb) range. Investigations of good milk samples and bad milk samples from alpine farms showed that ethanol, 3-methylbutan-1-ol, pentan-1-ol, and hexan-1-ol offered an increasing trend from good to bad milk samples. To enable more precise differentiation, further investigations with a higher sample size are necessary to reveal the feasibility of these markers within the complex matrix of raw milk. In the third step, these selected and literature-confirmed markers were presented to a commercially available sensor, run in a temperature-cycled operation and characterized by a self-developed system. When using ethanol, pentan-1-ol, and hexan-1-ol, a regression model with an accuracy of 42.9 ppb using partial least-squares regression (PLSR) analysis could be established, enabling such sensors to be used in raw-milk monitoring systems in the future.
- Article
(2927 KB) - Full-text XML
-
Supplement
(1186 KB) - BibTeX
- EndNote
Milk in general, and bovine milk in particular, is a valuable and nutritious food that is consumed worldwide. In Germany alone, 33 million t of cow's milk are produced each year, with an annual turnover of EUR 35.6 billion (MIV, 2023). After farming, raw bovine milk is distributed, collected, and transported by milk trucks fitted with chilled loading space and processed in dairies. In addition to liquid milk as product, milk is processed into other foods, including butter, buttermilk, cheese, and milk powder (MIV, 2023). As a sensitive raw product, fresh bovine milk is particularly susceptible to processes that affect its quality. Changes in the milk can be accompanied by the development of off-flavors and off-odors in the form of volatile organic compounds (VOCs) that arise due to chemical degradation, for example, by exposure to light and heat or by microbial spoilage (Forss, 1979; Beauchamp et al., 2014; Zardin et al., 2016) can indicate a quality impairment (Azzara and Campbell, 1992; Rashid et al., 2019). The early detection of volatile spoilage markers can therefore help to identify changes in quality and hence in safety as well as deliver valuable information on shelf life. Raw milk must be available in good condition to ensure the safety and quality of processed products. For example, an interruption of the cold chain can facilitate microbial growth, leading to a loss of quality and safety (BMEL, 2021; Roberts, 1993). Subsequently, the raw product must be discarded, which leads to increased losses, especially if good raw milk is mixed with spoiled raw milk in storage tanks. To indicate such deterioration of raw milk, automated systems that monitor the quality of raw milk on dairy farms, for example, when it is received or before it is transferred into storage tanks, can reduce associated losses. Currently, in Germany, a regular analysis of the raw milk by a certified laboratory is required by law (BMEL, 2021). In addition to measures such as determining bacterial counts, amongst others, a sensory assessment of the milk is often performed to assess odor impressions, representing the quality marker. As this method is subjective, it should be objectified by analytical standard methods to obtain a reliable quality evaluation criterion. A common analytical method to detect the characteristic off-odors arising from spoilage and degradation processes is laboratory-based gas chromatography (GC) coupled with mass spectrometry (MS) and olfactometry (O) (Friedrich and Acree, 1998; Schiano et al., 2017). However, the conventional GC requires a lot of supportive equipment, such as carrier gas sources and many others. It is usually laboratory-based, requires trained personnel and is cost- and time-intensive (roughly five to six figures and about 30 min for single analyses). In contrast, quality monitoring requires a system that is rather cost effective (roughly three to four figures maximum) and easy to use and can be readily installed in dairy facilities, on milk trucks, and in dairy farms. One way of realizing such a system is to utilize cheap and commercially available metal oxide semiconductor (MOS) gas sensors that have the ability to monitor VOCs in different application fields, such as air quality monitoring in indoor environments (Baur et al., 2018a; Koistinen et al., 2008; Geiss et al., 2011) or food quality and safety monitoring (Joppich et al., 2022; Koehne et al., 2023; García-González and Aparicio, 2010; Janssen et al., 2014; Bauersfeld et al., 2011). These sensors are known for being very sensitive (Baur et al., 2018a) but not highly selective for individual gaseous compounds as they measure oxidizing and reducing gases on the sensor surface (Wang et al., 2010). Research has focused on enhancing the selectivity of such sensors via temperature modulation and data processing steps (Baur et al., 2015). These modulations can be used for an enhanced VOC detection and hence for the raw-milk quality monitoring.
This paper presents a new concept for the development of a sensor system using the use case of raw-milk monitoring. The related methodological approach is designed to allow for transfer to other future applications. This concept consists of three steps, comprising compound identification (via GC-MS and GC-O), quantification (via proton transfer reaction–mass spectrometry; PTR-MS), and characterization (via a gas mixing apparatus; GMA). The aim of this approach is to find potential characteristic markers that are associated with the degradation and spoilage processes and to investigate the sensors' responses to these markers. An overview of the developed strategy is shown in Fig. 1.
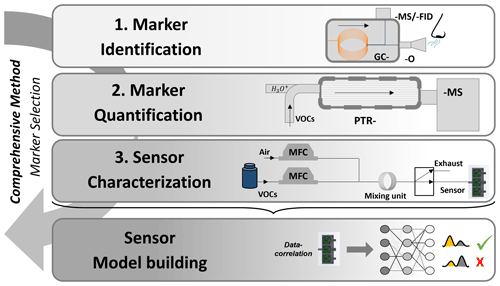
Figure 1Concept for a sensor system development. Identification of potential markers via gas chromatography–mass spectrometry, and gas chromatography–olfactometry (GC-MS and GC-O). Quantification of markers is performed via proton-transfer-reaction mass spectrometry (PTR-MS). Characterization is done via a gas mixing apparatus (GMA). Finally, the sensor model is created.
2.1 Marker identification (chromatographic analysis)
2.1.1 Milk samples and treatment
Raw-milk samples (good and bad milk) were provided as bulk samples by a local dairy from milk sourced from surrounding alpine farms. The differentiation between good and bad milk was made by the dairy based on internal quality parameters, including microbiological counts and odor impressions. Milk samples were defined as good when they had total viable counts of less than 1 000 000 in accordance to the German Federal Ministry of Food and Agriculture (BMEL, 2021) and exhibited no off-odor (determined by two experienced but non-sensory-trained human assessors according to the dairy's internal and industry standards). Samples were defined as bad when total viable counts were greater than 1 000 000 and an off-odor was present. Other combinations, such as increased total viable count without off-odor or vice versa, were not considered in this study. The raw-milk samples were kept at −20 °C during storage at the dairy, transportation, and storage at the research facilities until analysis. Prior to analysis, samples were defrosted for 45 min at 30 °C. To extract prospective volatile spoilage markers, the raw-milk samples were stirred with distilled dichloromethane (DCM; VWR International GmbH, Germany) at a 1:1 () ratio at 20 °C for 30 min. The resulting emulsion was then distilled by a solvent assisted flavour evaporation (SAFE) (Engel et al., 1999) unit at 50 °C and a pressure of 10−4 mbar according to a previously reported method (Debong et al., 2021; Engel et al., 1999). The distillate was separated in a separating funnel into organic and aqueous phases. The aqueous phase was washed three times by always adding 5 mL DCM and separating it again; the organic phases were combined and dried over sodium sulfate anhydrate (VWR International GmbH, Germany). The ensuing extract was concentrated to 100 µL by Vigreux distillation and micro-distillation.
2.1.2 Sample analysis
Gas chromatography–flame ionization detector/olfactometry (GC-FID/O) analyses of a 2 µL sample extract were performed on different TRACE GC Ultra systems (Thermo Fisher Scientific GmbH, Germany) equipped with either a DB-FFAP capillary column (30 m × 0.32 mm, 0.25 µm film thickness; Agilent Technologies, USA) or a DB-5 capillary column (30 m × 0.32 mm, 0.25 µm film thickness; Agilent Technologies, USA). A 1:1 split at the end of the column allowed for parallel detection by a FID and an odor detection port. For the high-resolution GC-MS measurements, a mass selective detector (MSD) quadrupole system (GC 7890 A coupled with an MS5970 C MSD; both by Agilent Technologies, USA) equipped with an MPS2 autosampler and a Cooled Injection System (CIS) 4 (both from Gerstel GmbH & Co. KG, Germany) and Xcalibur data system (version 1.4; Thermo Electron Corporation/Thermo Fisher Scientific) as data evaluation software were used for the identification of volatile compounds characterizing good milk and spoilage markers of bad milk. A DB-FFAP capillary column (30 m × 0.25 mm, 0.25 µm film thickness; Agilent Technologies, USA) or a DB-5 (30 m × 0.25 mm, 0.25 µm film thickness; Agilent Technologies, USA) was used for chromatographic separation with an injection volume of 1 µL. The temperature program for both measurements is listed within the Supplement (Sect. S1). Odorants were tentatively identified based on a comparison of the odor qualities as perceived at the sniffing port, and the retention indices were identified according to Van Den Dool and Kratz (1963) as determined from original substances on both DB-5 and DB-FFAP capillary columns together with their respective mass spectra in comparison to those of authentic reference compounds.
2.2 Marker quantification (PTR-MS measurements)
The description of the calibration procedure for the PTR-MS can be found within Sect. S2, and further details are given elsewhere (Fischer et al., 2013). For sampling, 5.00 ± 0.05 g milk was weighed into a 20 mL headspace vial. Subsequently, the vials were sealed and equilibrated for 30 min at 20 °C. An empty vial (n = 1) was incubated with the same procedure as a control sample. Sampling was carried out with a commercially available autosampler (Ionicon Analytik GmbH, Innsbruck, Austria) using filtered air as the carrier gas. Thereby, different carrier gas flow settings were applied (20 and 50 sccm on the first measurement day and 65 sccm on the second measurement day due to device-specific fluctuations on the second day which could not be decreased manually). A blank (filtered air) was measured directly before each sample. For each sample, a 5 mL headspace volume was injected with a constant flow (5 mL min−1 on the first and 16.25 mL min−1 on the second measurement day to adjust the gas mixing ratios for the different carrier gas flow settings) into the carrier gas stream. The milk samples were analyzed in a fivefold determination, respectively. As a control sample, an empty vial (n = 1) was measured on the second measurement day with a carrier gas flow of 65 sccm. The resulting gas stream was analyzed by PTR-ToF-MS 8000 (Ionicon Analytik GmbH, Innsbruck, Austria). The drift tube was set to 2.2 mbar, 80 °C, and 555 V, with an extraction voltage of 34 V, resulting in an electric field strength to buffer the gas number density ratio () of 105 Td (1 Td = 10−21 V m2). Each sample was analyzed with an acquisition rate of 1 Hz. The obtained data were processed with the PTR-MS Viewer software (version 3.4.5) provided by the PTR-ToF-MS manufacturer. For the purpose of quantification, the same signals used for calibration were monitored. The signal intensities in counts per second (cps) were corrected by the -specific transmission within the PTR-ToF-MS instrument and normalized to the primary ion signal ( 21 022, multiplier of 500), with a normalization factor of 107. The limits of detection (LODs) for the signal intensities given in normalized counts per second (ncps) ranged between 18 and 86 ncps; data below the LOD were excluded from further analysis (see further details in Sect. S2). The normalized signal intensities (ncps) were corrected by subtracting the blank signals and taking the gas dilution within the autosampler into account. Mean signal intensities were converted to gas-phase concentrations (given in parts per billion, ppb) using the sensitivities obtained from the calibrations. Thereby, 3-methylbutan-1-ol and pentan-1-ol were calculated as a sum parameter using the mean of both sensitivity values.
2.3 Gas sensor characterization
2.3.1 Gas sensors
Commercial gas sensors were characterized with respect to their response to the selected putative markers for raw-milk spoilage and degradation processes. In this paper, the results of the VOC sensor SGP40 (Sensirion AG, Switzerland) with four different sensor layers (layer 0–3; the exact composition of the layers is usually not published by the manufacturer, as for many commercially available sensors) and the respective different sensor responses are presented. In addition, the sensor BME688 (Bosch, Germany) was used to record temperature, humidity, and pressure during the measurement to monitor the stability of these parameters. Both sensors were integrated on separate sensor boards and measured in a specially designed sensor chamber housing all sensors. The sensor control was located on another board, which was connected to a computer via USB. The sensor chamber and the sensor control were based on the work of Baur et al. (2018b). The SGP40 was operated via temperature modulation developed by Baur et al. (2015) to increase the sensitivity of the sensors. For this purpose, a high-temperature plateau of 400 °C (for 15 s each) alternating with low-temperature plateaus of 150, 200, 250, and 300 °C (for 21 s each) was set for the SGP40, with a total cycle length of 144 s. The sensors were operated for 24 h under zero air exposure. The characteristic response behavior of each sensor was subsequently used for a regression analysis to develop a concentration prediction model. Therefore, the MATLAB-based open-access analysis software DAV3E (Lab for Measurement Technology, Saarland University, Germany) was used to analyze the data recorded by SGP40 and BME688 (Bastuck et al., 2018b).
2.3.2 Test gas generation
The test gases, ethyl acetate, pentan-1-ol, 3-methylbutan-1-ol, hexan-1-ol (from Sigma-Aldrich Chemie GmbH, Germany), ethanol, and acetic acid 99.99 % (from Carl Roth GmbH & Co. KG, Germany), were generated by the gas blender HovaCAL® 7836-VOC (IAS GmbH, Germany) at the respective concentrations of 50, 100, 200, and 500 ppb (except acetic acid, at 5, 10, 20, and 50 ppb). The gas blender incorporated three distinct manufacturing techniques: continuous syringe injection, capillary dosing, and thermal mass flow control in accordance with the DIN EN ISO 6145-1:2020-2 standard (ISO, 2020) to produce test gases at the desired concentrations. Thereby, the gas blender dosed certain amounts of the liquid components between 0.85 and 50 µL min−1 by syringe pumps over a capillary into an evaporator. The evaporator was constantly heated to 65 °C. The evaporated components were instantly diluted with 1500 mL min−1 of synthetic air to generate a pre-test gas concentration. The synthetic air was generated by the synthetic air generator CG15L (PEAK Scientific Instruments Ltd., United Kingdom). To achieve a test gas concentration at the parts per billion (ppb) level, the pre-test gas was further diluted to a factor of by a two-stage capillary dosing system. Each capillary dosing stage diluted 7.5 mL min−1 of the pre-test gas with 1500 mL min−1 of synthetic air. The amount of the liquid component was calculated by applying the ideal gas equation and the standard conditions of 1013.25 mbar and 0 °C. To avoid concentration errors caused by condensation effects in the gas blender, all lines inside the gas blender carrying the test gases were heated to 100 °C. The accuracy of this highly diluted test gas generation is specified by ±10 %. Over an additional humidifier, the diluted test gas with a constant humidity of 50 % RH was generated at a gas temperature of 23.5 °C. This test gas was adjusted to a flow rate of 1500 mL min−1 as defined by the gas blender. The sensor chamber was made of stainless steel and had a 1 × 1 mm gas flow channel in which the three sensors were mounted in a series. To ensure that the sensor chamber had as little dead volume as possible, the sensors were placed in recesses of 4 × 4 × 1.2 mm each. A 3 mm thick polytetrafluoroethylene (PTFE) plate was used for sealing, and the boards were screwed onto the plate with appropriate contact pressure. Commercially available PTFE tubing (Swagelok, USA) were used as gas lines and were connected to the sensor chamber and the gas blender. The absolute pressure in the sensor chamber was set to 1013 mbar using a vacuum pump behind the restriction (see Fig. 1, right side). In order to reduce the total gas flow rate of the gas mixer from 1500 to 200 NmL min−1 through the sensor chamber, appropriate flow restrictions were integrated into the setup. Two different lengths of in. stainless steel tubing were used for flow restriction.
3.1 Identification: GC-MS and GC-FID/O (identification of aroma compounds)
The characterization of the aroma compounds defining the off-odor was performed by GC-MS and GC-FID/O measurements. Identification was carried out by means of odor impression, retention index according to Van Den Dool and Kratz (1963) on two different capillary columns and mass spectra in comparison with reference substances. In the case of the spoiled milk, 3-methylbutan-1-ol (odor impression according to an internal database: malty, fruity, and solvent-like) was identified (retention index, RI, of DB-FFAP being 1198 and of DB-5 741). Other compounds found were pentan-1-ol (malty, almond-like, and solvent-like), hexan-1-ol (green-apple-like and almond-like), and acetic acid (vinegar-like). These substances could be confirmed using the literature (Azzara and Campbell, 1992; Rashid et al., 2019) as potential off-flavors and spoilage indicators and were chosen as potential markers for sensor characterization. In addition, hexanal, 2-methyl-1-propanol, limonene, nonanal, 2-ethylhexan-1-ol, butanoic acid, hexanoic acid, octanoic acid, methyl hexadecanoate, and decanoic acid were identified but not selected as potential markers due to their incompatibility with the requirements of the GMA (markers must be non-explosive and vaporizable). An overview of the entire chromatogram is given in Fig. 2a. Figure 2b shows, in comparison, the detailed excerpt of the first section of the chromatogram to display the more volatile compounds. As an extract obtained by the SAFE method was analyzed, both highly volatile and less volatile compounds are observable by this technique. Correspondingly, other substances with later retention times could not be detected by subsequent PTR-MS measurements in the headspace above the milk samples. The less volatile compounds were therefore not considered further. However, as some markers might be covered by the solvent peak in the course of the GC analysis (due to co-elution), the number of potential markers was also extended by ethanol (ethanolic odor impression) and ethyl acetate (glue-like and solvent-like) based on previous reports in the literature (Rashid et al., 2019; Natrella et al., 2020; Azzara and Campbell, 1992; Toso et al., 2002).
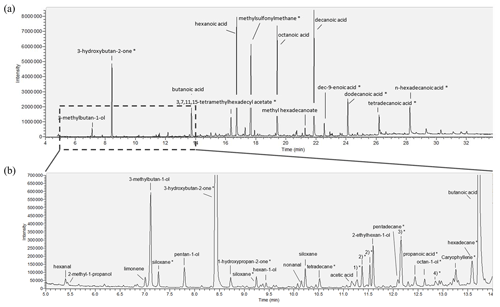
Figure 2(a) Entire chromatogram of milk measurements, measured on a DB-FFAP column (30 m × 0.32 mm, 0.25 µm film thickness, Agilent Technologies, USA). (b) Chromatogram excerpt of the tentatively identified potential volatile spoilage markers; the substances marked with an asterisk were proposed based on corresponding NIST database entries, and siloxane peaks presumably originate from the GC column. For better readability, numbers one to four are written out in full only here: 1)*: 2,6,10-trimethyltridecane; 2)*: 1-(1-methoxypropan-2-yloxy)propan-2-ol, which appears twice, identification not possible; 3)*: 1-(2-methoxypropoxy)propan-2-ol; and 4)*: 2-methylpropanoic acid.
3.2 Quantification of aroma active compounds: PTR-MS measurements
The measurements of each representative marker were repeated five times for eight good milk samples and five spoiled milk samples, respectively. The results of the individual markers did not show a clear result but nevertheless revealed a trend. A reason for that may be the complexity of the milk matrix. There are many influencing factors, such as feed or the individual animal's metabolism, which strongly influence the milk composition and hence may lead to different marker concentrations (Roberts, 1993; Bendall, 2001; Palmquist et al., 1993). In addition, there was a slight change in the aspiration rate of the PTR-MS (20 and 50 sccm on the first measurement day and 65 sccm on the second measurement day). Due to fluctuations within the device, the aspiration rate on the second day could not be decreased to match the values on the first day of measurement. However, as a trend could be seen, the PTR-MS data of both good and spoiled milk samples were averaged to obtain a mean value with the corresponding standard deviation.
Outliers were defined as a deviation of more than 2 times the standard deviation from the mean. As they occurred within four measurements, the corresponding mean values were calculated and displayed with and without the outliers. They occurred in the good milk samples in one measurement of ethanol, one measurement of hexan-1-ol, one measurement of acetic acid, and one measurement of ethyl acetate. A reason for their appearance may be possible storage or transportation problems or problems during the thawing and freezing processes, causing microbiological growth and thus slightly deteriorating the previously good milk samples (Roberts, 1993; BMEL, 2021). Nevertheless, with and without these outliers, a trend can be observed for the fragments of ethanol, hexan-1-ol, 3-methyl-1-butanol, pentan-1-ol, and acetic acid, which makes them suitable as possible markers. It is worth mentioning that PTR reactions of butanoic acid with H3O+ result in 89 060 and 71 049 as major ions according to Smith et al. (2011). As ethyl acetate ( 89 060) and the main fragment ions of 3-methyl-butan-1-ol and pentan-1-ol ( 71 086) have the same or similar values, an overlap might occur. This overlap can influence the actual measured values and falsify them for the respective markers. A consideration of the total signal (sum) of these markers for further model building instead of individual markers may therefore be a preferred solution for the raw-milk monitoring. However, the individual markers with the corresponding concentrations from the PTR-MS measurements are analyzed in a first approach below. An overview of the PTR-MS results (mean and standard deviation with and without outliers) is given in Fig. 3. The corresponding mean concentration values are shown in Table 1. An overview of all single measurements and their respective values is provided within the Supplement (Sect. S2 on PTR-MS measurements).
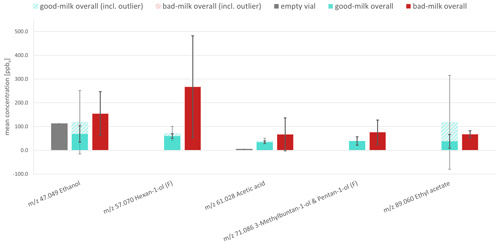
Figure 3Mean concentrations of potential markers for raw-milk spoilage, measured via PTR-MS headspace for good milk samples (green) and spoiled milk samples (red). Striped bars are mean values including outliers, and filled bars are mean values without outliers. Error bars in grey include outliers, while error bars in black exclude outliers. F denotes the detected ion fragment (see Sect. S2).
3.3 Characterization of gas sensors
3.3.1 Calculation of flow restrictions
Flow restrictions were used to set a flow of 200 mL min−1 through the sensor chamber according to Bastuck et al. (2018a) and Richter et al. (2013) as the GMA was producing a constant flow of 1500 mL min−1. To determine the required flow restrictions, a five-step approach based on the law of Hagen–Poiseuille (Eq. 1) was used here. For the sake of simplicity, the form for incompressible fluids was used, fulfilling the requirements of a first approximation for small pressure differences. Initially, the highest restriction needs to be identified within the actual setup. Therefore, Eq. (1) can be considered Ohm's law, which gives the equation for flow resistance according to electrical resistance (Eq. 2).
Δp is the difference between the inlet and outlet pressure, which is therefore comparable with the voltage, U, in Ohm's law. For the dynamic viscosity, η, values for nitrogen at 22 °C are assumed as nitrogen serves as the carrier gas within the gas mixing apparatus and as experiments are conducted at a controlled laboratory atmosphere of 22 °C. In the setup for the gas sensor characterization chosen here (see Fig. 1), the sensor chamber for the three sensors has the smallest volume. Hence, it is the highest flow restriction to start with. Subsequently, this restriction needs to become negligible. Hence, an at least 10-fold higher resistance needs to be introduced as an actual flow restriction, Rres, that can be integrated into the setup. In the next step, the length of the new resistance needs to be determined through the Hagen–Poiseuille resistance (Eq. 3).
Therefore, a in. Swagelok tube with a radius of 0.54 mm was chosen as the restriction, and a length of 798.49 mm was calculated. In the fourth step, the inlet pressure needs to be determined via Eq. (4) and a desired split for the gas streams needs to be chosen. Therefore, the split flow rate is calculated so that finally 200 mL min−1 of the total flow passes through the sensor chamber, while the rest (here 1.3 L) passes through a bypass. Since the system is open at the back end, an approximate outlet pressure of 1013.25 hPa is assumed. The calculated inlet pressure was 1027.36 hPa.
The length of the second restriction needed to be determined in the fifth and final step in such a way that the rest of the total stream originating from the GMA (1.3 L) flows through the bypass. Therefore, a in. Swagelok tube was used again, and the length was calculated according to Eq. (1), revealing a desired length of 122.85 mm. The final setup with the calculated flow restrictions is presented schematically in Fig. 4.
3.3.2 Sensor test gas measurements
To evaluate the temperature-cycled data, quasi-static signals were generated in a first approach by plotting a signal curve at one point in the temperature modulation cycle to see the sensor response in general. Thereby, the sensor signal is proportional to the logarithm of the resistance of the sensing material and hence to the conductance as depicted in the manufacturer's specifications (Sensirion, 2023).
As an example, the curve after 105 s of cycle time was selected. In the cycle progression, the progression between the high- and low-temperature phases can be followed as the high-temperature plateau is always about the same height in the sensor's response, while the low-temperature steps (150–300 °C in 50 °C steps) differ in their sensor response height (Fig. 5a, left column). The SGP40 showed a concentration-dependent response in the ppb range of 50–500 ppb for the various substances, corresponding to sensor layer 2 (Fig. 5a, middle and right). For the general sensor response, a mean value is calculated during an 8 h zero airflow (R0) and subtracted from the measured value of the measurements (), and as the signal is logarithmic, it amounts to
Layer 2 was chosen to be shown here exemplarily as it offers the strongest signal response (Fig. 5b and c; an overview of other layers can be found in Sect. S3).
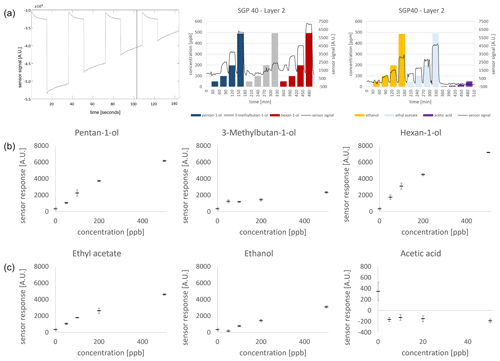
Figure 5Sensor signal and sensor response; (a) exemplary data extract. On the left is a randomly chosen temperature cycle with set point 105 s (black bar). In the middle and right columns is the quasi-static signal as a relative sensor response of layer 2 to all measured marker substances at the set point of 105 s. (b) Sensor response (log (R)−log (R0)) of pentan-1-ol (left), 3-methylbutan-1-ol (middle), and hexan-1-ol (right). (c) Sensor response (log (R)−log (R0)) of ethyl acetate (left), ethanol (middle), and acetic acid (right).
The individual sensor layer responses, namely, 0–3, from the four different sensor layers of the SGP40 with the respective concentrations supplied by the GMA (bar graphs) and the corresponding sensor response over time (line graphs) are not shown as further data evaluation includes all layers automatically. The BME688 showed a stable and constant progression of the humidity (50 %) and temperature (23 °C). The pressure was set to 1013 mbar within the sensor chamber and revealed a constant progression as well. An overview of the parameters measured with the BME688 can be found within the Supplement (see Sect. S4). It is noticeable that at this randomly chosen point, the signal response for 3-methylbutan-1-ol showed a low sensor response in contrast to hexan-1-ol, which yielded the strongest intensity and thus the clearest response (Table 2 and Fig. 5b). Compared to pentan-1-ol, hexan-1-ol was found to have a slightly stronger signal. For pentan-1-ol, the signal response is very low in the 50 ppb range, whereas for hexan-1-ol, a clear response can be observed from the 50 ppb level onwards. Above 100 ppb, clear sensor responses were observed for pentan-1-ol and hexan-1-ol. 3-Methylbutan-1-ol showed a clear sensor response only at a concentration of 500 ppb. A differentiation between 50, 100, and 200 ppb was not possible. Ethanol showed a clear signal response from a concentration of 100 ppb, whereas ethyl acetate gave a clear signal already from a concentration of 50 ppb (Fig. 5a, middle and right columns). Acetic acid was measured diluted by a factor of 10 based on the respective PTR-MS results, which is why a signal was not clearly visible here. An overview of the sensor responses is given in Fig. 5 and Table 2.
As MOS sensors are found to specifically react to functional groups of molecules (Baur et al., 2021), building a model containing all alcoholic compounds, namely 3-methylbutan-1-ol, hexan-1-ol, pentan-1-ol, and ethanol, was considered to detect the raw-milk quality in the next step (Fig. 6). However, in order to consider a complete data evaluation of the sensor response behavior and to obtain a quantification model for the potential markers, DAV3E, an analysis software explicitly suitable for temperature-cyclic data, was used (Bastuck et al., 2018b). For this purpose, the raw sensor data from all four sensor layers of the SGP40 were first multiplied by a factor of −1 to account for the conductance of the sensor (Baur et al., 2015). After an appropriate assignment of the GMA concentrations to the sensor signals, a feature extraction was performed. For this purpose, the low-temperature sections of the cycles were divided into five blocks of 4 s each. The low-temperature plateaus were chosen because of the signal run-in behavior after high temperatures, which is more sensitive to VOCs than the application of the high-temperature plateaus (Baur et al., 2017a, b, 2015). The slope and mean were determined from the resulting intervals. With four different sensor layers, each with four partial temperature plateaus; their subdivision into five intervals each; and the two features, slope and mean values, a total of 160 features were used to build the model. The next step was to run a PLSR (partial least-squares regression) for a variable number of components. The data were then validated using a k-fold analysis (10 folds, two iterations). The model was tested using a holdout analysis (20 % holdout, one iteration). The data considered in the test were randomly selected by the software and therefore not used to build the model. As the consideration of four PLSR components gave the best overall ratio between validation and test (see Sect. S5), only the results of the PLSR model with four components were considered in the following (see Fig. 6).
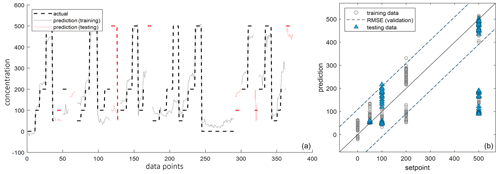
Figure 6Prediction (a) and regression (b) of alcoholic substances within the parts per billion (ppb) range measured with an SGP40-MOS sensor (Sensirion AG, Switzerland).
As can be seen in Fig. 6, the regression and the prediction model revealed here offered a bad solution (four components; RMSE > 100 ppb). For the set-point of 500 ppb in particular, a proper prediction was not possible as predicted values were around 100 ppb. Hence, the model was unsuitable for using all alcohols as potential markers for the measurement of bad milk. A possible reason could be the inclusion of 3-methylbutan-1-ol. It shows a very low sensor response in the quasi-static signal that is roughly about the same signal height as hexan-1-ol shows at 100 ppb. A potential cause for this could be the filter element of the SGP40. It is located in front of the sensor surface and is supposed to keep dust and possibly siloxanes away from the sensor surface and thus counteract sensor poisoning. As 3-methylbutan-1-ol is sterically branched, there could also be an unintended filter effect, hindering it from reaching the sensor surface (Sensirion, 2023; Schultealbert et al., 2021). Therefore, in the next step, the data analysis was repeated but without the 3-methylbutan-1-ol data. The parameters used were set to be exactly equal as they were in the first analysis. The results obtained show a better regression (four components; RMSE of 42.9 ppb) where the set points fit the predictions (Fig. 7). However, there are still some outliers, especially at 50 and 100 ppb, which could make prediction difficult in the lower concentration ranges and which need to be considered in future. To conclude, the model is capable of quantifying the marker substances as a set of alcohols, without 3-methylbutan-1-ol, and can therefore be used to detect potential markers of spoilage and degradation processes. Before bringing it to real sample measurements, further steps are required to ensure a reliable statement about the quality status of the milk.
This paper presents a new and comprehensive method for the development of a sensor system using the use case of raw-milk monitoring. This method is designed to be generalizable and can be transferred to other fields of application in the future (Zeh et al., 2022). It consisted of three steps. First, a laboratory analysis was performed using GC-MS and GC-FID/O to tentatively identify markers which are specific for the off-odors of degradation and spoilage processes of raw milk. Thereby, GC-MS and GC-FID/O measurements revealed 3-methylbutan-1-ol, hexan-1-ol, pentan-1-ol, and acetic acid as potential markers for raw-milk spoilage, which could be confirmed by the literature (Azzara and Campbell, 1992; Rashid et al., 2019). Since other highly volatile potential markers can be lost in the solvent peak of the chromatogram, the markers ethanol and ethyl acetate known from the literature were added (Rashid et al., 2019; Azzara and Campbell, 1992; Natrella et al., 2020; Toso et al., 2002). In the second step, PTR-MS measurements revealed relevant target concentration areas for the marker substances in the ppb range. In addition, a trend between good and bad milk samples could be found for ethanol, pentan-1-ol, hexan-1-ol, 3-methylbutan-1-ol, and acetic acid. This trend could be observed when calculating the mean values for the markers in the good milk samples and in the bad milk samples. Using single-sample analysis, it was difficult to obtain the distinct quality of the milk, which is likely due to the complexity of the milk matrix (Friedrich and Acree, 1998) and the numerous influencing factors, such as feed or individual animal properties (Palmquist et al., 1993; Bendall, 2001; Roberts, 1993) and also freezing, storing, and transportation condition issues. Therefore, further studies with larger sample sizes are needed to determine the unambiguous usability of markers with respect to the complex matrix of raw milk for quality monitoring. In the third step, a commercially available MOS sensor was characterized with respect to its detection properties towards the potential markers mentioned above. It was shown that the SGP40 used was able to respond to the selected spoilage markers in the ppb range. For this purpose, a regression analysis was performed with the data of all alcohols (ethanol, pentan-1-ol, hexan-1-ol, and 3-methylbutan-1-ol as confirmed by the PTR-MS measurements) to determine whether an alcohol signal was suitable for the detection of spoiled milk. The model was validated using a k-fold analysis and then tested with a holdout analysis. The model obtained was initially inappropriate to detect the potential markers (RMSE > 100 ppb). It was suspected that 3-methylbutan-1-ol was retained by the filter unit of the sensor due to steric hindrance and therefore showed a weaker signal than other alcohols at the same concentration (Schultealbert et al., 2021; Sensirion, 2023). Thus, the data for 3-methylbutan-1-ol were excluded for the second modeling. The resulting model showed reasonable results regarding the marker detection, and the SGP40 sensor revealed a good response. We could demonstrate that an important group of potential markers can be quantified with an accuracy of 42.9 ppb using PLSR (partial least-squares regression) analysis. Hence, the SGP40 is principally suitable for the detection of raw-milk quality.
In the future, the influence of the filter unit on the 3-methylbutan-1-ol signal should be further investigated, possibly by removing the membrane. In addition, a larger range of good and bad milk samples with varying characteristics from farms needs to be collected to reveal distinct quality markers in the complex milk matrix (Bendall, 2001; Friedrich and Acree, 1998; Roberts, 1993) within the first two steps. Furthermore, the sensor responses are to be checked for actual disturbance variables and cross-sensitivities. For this purpose, the respective spoilage markers can be mixed and measured together with interfering variables such as traces of hydrogen or carbon monoxide as ubiquitous gases. If the results are unambiguous, the sensor can be tested on real milk samples using the corresponding quality detection model. If necessary, a simple chromatographic separation unit in the form of a short fused capillary column can be installed to separate the analyte mixture into the individual analytes in the case of signal overlap as reported elsewhere (Koehne et al., 2023; Bauersfeld et al., 2009; García-González and Aparicio, 2010). For a final measurement device, more statistical power with different milk samples during different seasons is needed for a comprehensive data analysis. Finally, a comprehensive method for sensor system development for the characterization of MOS sensors could be presented with this study. In the future, this concept can be used for a sensor system development (Zeh et al., 2022) for raw-milk control at dairy farms with the specific aim to monitor the condition of raw milk and to thus ensure the safety and quality of the milk. We expect that such a sensor system, when applied at a large scale, will specifically bring support in the daily production of milk and milk products in small- and medium-size enterprises and farms and will thus contribute to high-quality production and resilience in local dairy industries.
All data can be provided by the corresponding authors on reasonable request.
The supplement related to this article is available online at: https://doi.org/10.5194/jsss-13-263-2024-supplement.
MK, MH, TS, and GZ: conceptualization. MK, MH, and KA: investigation. MK, MH, KA, and AS: formal analysis. MK and MH: methodology. GZ, TS, ST, and AB: supervision. MK, MH, KA, and AS: writing (original draft preparation). MK, MH, TS, GZ, KA, AS, ST, and AB: writing (review and editing).
The contact author has declared that none of the authors has any competing interests.
Publisher’s note: Copernicus Publications remains neutral with regard to jurisdictional claims made in the text, published maps, institutional affiliations, or any other geographical representation in this paper. While Copernicus Publications makes every effort to include appropriate place names, the final responsibility lies with the authors.
This work was carried out as part of the research network SHIELD – Sichere heimische (Bio-)Lebensmittel durch sensorische Detektions-verfahren (SHIELD – Safe domestic (organic) food through sensory detection methods) and funded by the Bayerische Forschungsstiftung (Bavarian Research Foundation). The preliminary work on the triplet sensor chamber was funded by the Bayerisches Staatsministerium für Wirtschaft, Landesentwicklung und Energie (Bavarian State Ministry of Economic Affairs, Regional Development and Energy) as part of the project Campus der Sinne (Campus of the Senses).
We would also like to thank the Lab for Measurement Technology at Saarland University and in particular Oliver Brieger and Johannes Amann for their kind support in implementing the data analysis with the open-source software DAV3E and Stephanie Fechner from the Fraunhofer Institute for Process Engineering and Packaging IVV for her support with the PTR-MS measurements.
This research has been supported by the Bayerische Forschungsstiftung (grant no. AZ-1491-20) and the Bayerische Staatsministerium für Wirtschaft, Landesentwicklung und Energie (grant no. 20-3410-2-14-3).
This paper was edited by Michele Penza and reviewed by two anonymous referees.
Azzara, C. D. and Campbell, L. B.: Off-Flavors of Dairy Products, Dev. Food Sci., 28, 329–374, https://doi.org/10.1016/B978-0-444-88558-6.50018-0, 1992.
Bastuck, M., Baur, T., Richter, M., Mull, B., Schütze, A., and Sauerwald, T.: Comparison of ppb-level gas measurements with a metal-oxide semiconductor gas sensor in two independent laboratories, Sensor. Actuat. B-Chem., 273, 1037–1046, https://doi.org/10.1016/j.snb.2018.06.097, 2018a.
Bastuck, M., Baur, T., and Schütze, A.: DAV3E – a MATLAB toolbox for multivariate sensor data evaluation, J. Sens. Sens. Syst., 7, 489–506, https://doi.org/10.5194/jsss-7-489-2018, 2018b.
Bauersfeld, M.-L., Peter, C., Wöllenstein, J., Bücking, M., Bruckert, J., and Steinhanses, J.: B5.4 - Gas sensor array for low-cost gas chromatography in food industry processes, in: SENSOR+TEST Conferences 2009, Congress Center Nürnberg, Nürnberg, Germany, 25–28 October 2009, Proceedings SENSOR 2009, 1, 245–250, https://doi.org/10.5162/sensor09/v1/b5.4, 2009.
Bauersfeld, M.-L., Bucking, M., Bruckert, J., and Wollenstein, J.: Miniaturised gas chromatographic system with metal oxide gas sensor array for fast detection of off-flavors, in: 2011 16th International Solid-State Sensors, Actuators and Microsystems Conference, Beijing, China, 5–9 June 2011, IEEE, 2086–2089, https://doi.org/10.1109/TRANSDUCERS.2011.5969262, 2011.
Baur, T., Schütze, A., and Sauerwald, T.: Optimierung des temperaturzyklischen Betriebs von Halbleitergassensoren, tm - Technisches Messen, 82, 187–195, https://doi.org/10.1515/teme-2014-0007, 2015.
Baur, T., Schütze, A., and Sauerwald, T.: A4.2 - Detection of short trace gas pulses, in: Proceedings Sensor 2017, AMA Conferences 2017, Nürnberg, Germany, 30 May–1 June 2017, 87–91, https://doi.org/10.5162/sensor2017/A4.2, 2017a.
Baur, T., Schütze, A., and Sauerwald, T.: Detektion von kurzen Gaspulsen für die Spurengasanalytik, tm - Technisches Messen, 84, 88–92, https://doi.org/10.1515/teme-2017-0035, 2017b.
Baur, T., Schultealbert, C., Schütze, A., and Sauerwald, T.: Novel method for the detection of short trace gas pulses with metal oxide semiconductor gas sensors, J. Sens. Sens. Syst., 7, 411–419, https://doi.org/10.5194/jsss-7-411-2018, 2018a.
Baur, T., Schultealbert, C., Schütze, A., and Sauerwald, T.: Device for the detection of short trace gas pulses, tm - Technisches Messen, 85, 496–503, https://doi.org/10.1515/teme-2017-0137, 2018b.
Baur, T., Amann, J., Schultealbert, C., and Schütze, A.: Field Study of Metal Oxide Semiconductor Gas Sensors in Temperature Cycled Operation for Selective VOC Monitoring in Indoor Air, Atmosphere, 12, 647, https://doi.org/10.3390/atmos12050647, 2021.
Beauchamp, J., Zardin, E., Silcock, P., and Bremer, P. J.: Monitoring photooxidation-induced dynamic changes in the volatile composition of extended shelf life bovine milk by PTR-MS, J. Mass Spectrom., 49, 952–958, https://doi.org/10.1002/jms.3430, 2014.
Bendall, J. G.: Aroma compounds of fresh milk from New Zealand cows fed different diets, J. Agr. Food Chem., 49, 4825–4832, https://doi.org/10.1021/jf010334n, 2001.
BMEL (Bundesministerium für Ernährung und Landwirtschaft/Federal Ministry of Food and Agriculture): Verordnung zur Förderung der Güte von Rohmilch (Rohmilchgüteverordnung - RohmilchGütV): RohmilchGütV (Ordinance on the Promotion of the Quality of Raw Milk (Raw Milk Quality Ordinance - RohmilchGütV): RohmilchGütV), 2021.
Debong, M. W., N'Diaye, K., Owsienko, D., Schöberl, D., Ammar, T., Lang, R., Buettner, A., Hofmann, T., and Loos, H. M.: Dietary Linalool is Transferred into the Milk of Nursing Mothers, Mol. Nutr. Food Res., 65, e2100507, https://doi.org/10.1002/mnfr.202100507, 2021.
Engel, W., Bahr, W., and Schieberle, P.: Solvent assisted flavour evaporation – a new and versatile technique for the careful and direct isolation of aroma compounds from complex food matrices, Eur. Food Res. Technol., 209, 237–241, https://doi.org/10.1007/s002170050486, 1999.
Fischer, L., Klinger, A., Herbig, J., Winkler, K., Gutmann, R., and Hansel, A.: The LCU: versatile trace gas calibration, in: 6th International Conference on Proton Transfer Reaction Mass Spectrometry and its Applications Sölden-Obergurgel, Austria, 3–8 February 2013, 192–194, ISBN 9783902811912, 2013.
Forss, D. A.: Mechanisms of formation of aroma compounds in milk and milk products, J. Dairy Res., 46, 691–706, https://doi.org/10.1017/s0022029900020768, 1979.
Friedrich, J. E. and Acree, T. E.: Gas Chromatography Olfactometry (GC/O) of Dairy Products, Int. Dairy J., 8, 235–241, https://doi.org/10.1016/S0958-6946(98)80002-2, 1998.
García-González, D. L. and Aparicio, R.: Coupling MOS sensors and gas chromatography to interpret the sensor responses to complex food aroma: Application to virgin olive oil, Food Chem., 120, 572–579, https://doi.org/10.1016/j.foodchem.2009.09.097, 2010.
Geiss, O., Giannopoulos, G., Tirendi, S., Barrero-Moreno, J., Larsen, B. R., and Kotzias, D.: The AIRMEX study - VOC measurements in public buildings and schools/kindergartens in eleven European cities: Statistical analysis of the data, Atmos. Environ., 45, 3676–3684, https://doi.org/10.1016/j.atmosenv.2011.04.037, 2011.
ISO: Gas analysis - Preparation of calibration gas mixtures using dynamic methods: Part 1: General aspects (ISO 6145-1:2019); German version EN ISO 6145-1:2019, DIN German Institute for Standardization, DIN EN ISO 6145-1:2020-02, 71.040.40 Chemische Analyse, Beuth Verlag GmbH, Berlin, https://doi.org/10.31030/3069640, 2020.
Janssen, S., Schmitt, K., Blanke, M., Bauersfeld, M. L., Wöllenstein, J., and Lang, W.: Ethylene detection in fruit supply chains, Philos. T. Roy. Soc. A, 372, 20130311, https://doi.org/10.1098/rsta.2013.0311, 2014.
Joppich, J., Su, Z., Marschibois, M., Karst, K., Bur, C., and Schütze, A.: 2.3 – MOS Sensors for Spoilage Detection of Milk using GC-MS and Human Perception as Reference, in: 16. Dresdner Sensor-Symposium 2022, Dresden, Germany, 5–7 December 2022, Vorträge, 17–22, https://doi.org/10.5162/16dss2022/2.3, 2022.
Koehne, M., Schmidt, C., Singh, S., Grasskamp, A., Sauerwald, T., and Zeh, G.: Development of a gas chromatography system coupled to a metal-oxide semiconductor (MOS) sensor, with compensation of the temperature effects on the column for the measurement of ethene, J. Sens. Sens. Syst., 12, 215–223, https://doi.org/10.5194/jsss-12-215-2023, 2023.
Köhne, M., Zeh, G., Sauerwald, T., Henfling, M., Amtmann, K., Büttner, A., and Trupp, S.: P28 – Charakterisierung kommerzieller Gassensoren zur Detektion von Verderbsmarkern in Kuhmilch, in: 16. Dresdner Sensor-Symposium 2022, Dresden, Germany, 5–7 December 2022, Poster, 160–165, https://doi.org/10.5162/16dss2022/P28, 2022.
Koistinen, K., Kotzias, D., Kephalopoulos, S., Schlitt, C., Carrer, P., Jantunen, M., Kirchner, S., McLaughlin, J., Mølhave, L., Fernandes, E. O., and Seifert, B.: The INDEX project: executive summary of a European Union project on indoor air pollutants, Allergy, 63, 810–819, https://doi.org/10.1111/j.1398-9995.2008.01740.x, 2008.
MIV (Milch Industrie Verband/Milk Industry Association): Fakten Milch: Milch und mehr - die deutsche Milchwirtschaft auf einen Blick, Infomrationsbroschüre des Milchindustrie-Verbandes e.V., Berlin, https://milchindustrie.de/marktdaten/produktion/, last access: 8 October 2023.
Natrella, G., Gambacorta, G., and Faccia, M.: Volatile organic compounds throughout the manufacturing process of Mozzarella di Gioia del Colle PDO cheese, Czech J. Food Sci., 38, 215–222, https://doi.org/10.17221/129/2020-CJFS, 2020.
Palmquist, D. L., Beaulieu, A. D., and Barbano, D. M.: Feed and animal factors influencing milk fat composition, J. Dairy Sci., 76, 1753–1771, https://doi.org/10.3168/jds.S0022-0302(93)77508-6, 1993.
Rashid, A., Javed, I., Rasco, B., Sablani, S., Ayaz, M., Ali, M., Abdullah, M., Imran, M., Gondal, T., Afzal, M., Atif, M., Salehi, B., Rodrigues, C., Sharifi-Rad, J., and Martins, N.: Measurement of Off-Flavoring Volatile Compounds and Microbial Load as a Probable Marker for Keeping Quality of Pasteurized Milk, Appl. Sci., 9, 959, https://doi.org/10.3390/app9050959, 2019.
Richter, M., Jann, O., Horn, W., Pyza, L., and Wilke, O.: System to generate stable long-term VOC gas mixtures of concentrations in the ppb range for test and calibration purposes, Gefahrstoffe, Reinhaltung der Luft = Air quality control, Springer-VDI-Verlag, 73, 103–106, https://opus4.kobv.de/opus4-bam/frontdoor/index/index/docId/27903 (last access: 26 June 2023), 2013.
Roberts, H. A.: Raw milk quality - milk flavor, Kansas Agricultural Experiment Station Research Reports, 57–60, https://doi.org/10.4148/2378-5977.2966, 1993.
Schiano, A. N., Harwood, W. S., and Drake, M. A.: A 100-Year Review: Sensory analysis of milk, J. Dairy Sci., 100, 9966–9986, https://doi.org/10.3168/jds.2017-13031, 2017.
Schultealbert, C., Amann, J., Baur, T., and Schütze, A.: Measuring Hydrogen in Indoor Air with a Selective Metal Oxide Semiconductor Sensor, Atmosphere, 12, 366, https://doi.org/10.3390/atmos12030366, 2021.
Sensirion: SGP40 – VOC sensor for HVAC and air quality applications: Datasheet SGP40, https://sensirion.com/products/catalog/SGP40, last access: 30 August 2023.
Smith, D., Chippendale, T. W., and Španěl, P.: Selected ion flow tube, SIFT, studies of the reactions of H3O+, NO+ and O with some biologically active isobaric compounds in preparation for SIFT-MS analyses, Int. J. Mass Spectrom., 303, 81–89, https://doi.org/10.1016/j.ijms.2011.01.005, 2011.
Toso, B., Procida, G., and Stefanon, B.: Determination of volatile compounds in cows' milk using headspace GC-MS, J. Dairy Res., 69, 569–577, https://doi.org/10.1017/S0022029902005782, 2002.
Van Den Dool, H. and Kratz, P. D.: A generalization of the retention index system including linear temperature programmed gas-liquid partition chromatography, J. Chromatogr., https://worldveg.tind.io/record/1766/ (last access: 19 March 2024), 1963.
Wang, C., Yin, L., Zhang, L., Xiang, D., and Gao, R.: Metal oxide gas sensors: sensitivity and influencing factors, Sensors-Basel, 10, 2088–2106, https://doi.org/10.3390/s100302088, 2010.
Zardin, E., Silcock, P., Siefarth, C., Bremer, P. J., and Beauchamp, J.: Dynamic changes in the volatiles and sensory properties of chilled milk during exposure to light, Int. Dairy J., 62, 35–38, https://doi.org/10.1016/j.idairyj.2016.07.005, 2016.
Zeh, G., Koehne, M., Grasskamp, A., Haug, H., Singh, S., and Sauerwald, T.: Towards Artificial Intelligent Olfactory Systems, in: 2022 IEEE International Symposium on Olfaction and Electronic Nose (ISOEN), Aveiro, Portugal, 29 May–1 June 2022, IEEE, 1–2, https://doi.org/10.1109/ISOEN54820.2022.9789600, 2022.